How the DeepMind Montreal Team is Contributing to Solving Intelligence
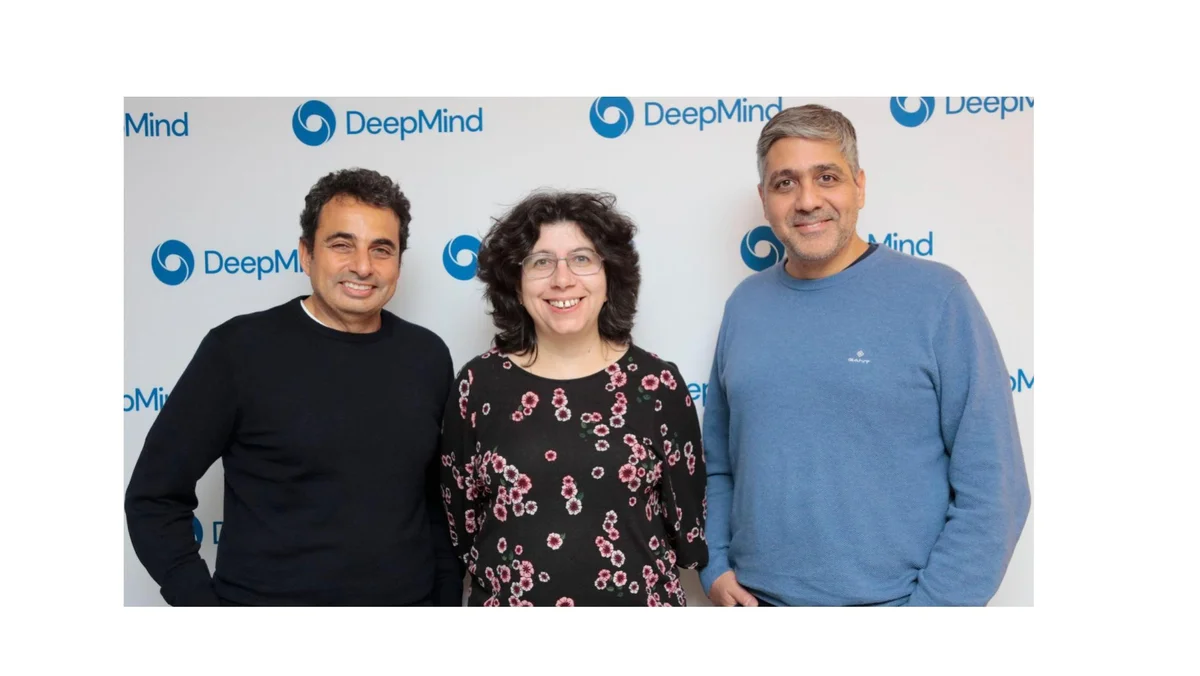
Today's blog post is guest-authored by Shibl Mourad, Research Engineering Director for DeepMind. As 2022 comes to a close, DeepMind celebrates 5 years of commitment to research and innovation. Read on to hear how DeepMind established roots in Canada and how it's pushing the boundaries of intelligence.
DeepMind, a subsidiary of Alphabet Inc., is a scientific discovery company, committed to ‘solving intelligence to advance science and benefit humanity.’ Solving intelligence requires a diverse and interdisciplinary team working closely together – from scientists and designers, to engineers and ethicists – to pioneer the development of advanced artificial intelligence.
***
As we celebrate DeepMind Montreal’s 5th anniversary, I remember my first meeting with the DeepMind team and how it forever changed the course of my career.
DeepMind's mission resonates as loudly with me today as it did five years ago when I first heard it: "Solving intelligence, to advance science and benefit humanity." This mission constitutes one of the most ambitious scientific endeavors in history, and yet DeepMind had already achieved so much in their pursuit of it.
For example, giving the scientific community free and open access to AlphaFold has transformed biology, aiding countless more scientists to open up completely new solutions to tackle some of our biggest global challenges. We’ve also made progress in building AI that communicates in a helpful, correct and harmless way through our dialogue agent Sparrow, which reduces the risk of unsafe and inappropriate answers.
As Google Montreal Site Lead at the time, I had been following DeepMind’s progress and I was eager to convince them to start a research lab in the city. What I did not expect was to come out of that meeting so inspired, that I would decide to jump ship and join them.
With the support of many colleagues, and in partnership with Doina Precup, a world leading pioneer in reinforcement learning, we established DeepMind Montreal in October 2017. Since then, we have grown into a diverse team of research scientists and engineers. The team has made major contributions to DeepMind's mission and the scientific advancement of AI, contributing to over 45 scientific papers in leading Machine Learning conferences and peer reviewed journals. These include work in diverse topics such as: Infection detection, collaborative AI, ethical and social risks, and theorem proving (detailed list at the end of this article).
One thing I am very proud of is that we reversed the ‘brain drain‘ of the best talents leaving Montreal and Canada to work in places like Silicon Valley. Our researchers come not only from Montreal but from all over the world. Montreal is now a sponge, attracting world class researchers from all over the world: US, India, Croatia, Hungary, Romania, UK, France, Russia, and other countries.
Montreal's thriving ecosystem of AI researchers, startups and leading companies, combined with major public investments in AI development and adoption had established the city as a world-class artificial intelligence hub. Montréal is also home to world-renowned researchers and institutions, and is home to Mila, the world’s largest academic research lab specialized in deep learning and reinforcement learning with close to 900 researchers.
Looking back on these past 5 years, DeepMind's commitment and contributions to the field of science have been outstanding but more importantly its pursuit of ensuring that AI is built in a fair and responsible manner that contributes positively to the world.
Looking ahead to the next 5 years, DeepMind will continue to invest in Montreal, nurture our world-leading expertise in reinforcement learning and abstract reasoning for AI, and contribute to and benefit from this city’s great AI community and ecosystem.
I am very excited to be part of this journey and of what the future holds for us: building AI safely, with creativity, compassion, and in a responsible manner.
A selection papers DeepMind Montréal collaborated on:
- A Clinically Applicable Approach to the Continuous Prediction of Future Acute Kidney Injury
- Symbolic Behaviour in Artificial Intelligence
- Cooperative AI: machines must learn to find common ground
- Reward is Enough
- RLDS: an Ecosystem to Generate, Share and Use Datasets in Reinforcement Learning
- Learning Robust Real-Time Cultural Transmission without Human Data
- Emergent Communication at Scale
- Ethical and social risks of harm from Language Models
- Learning to cooperate: Emergent communication in multi-agent navigation
- Improving alignment of dialogue agents via targeted human judgements
- AndroidEnv: A Reinforcement Learning Platform for Android
- Proving Theorems using Incremental Learning and Hindsight Experience Replay
- Challenges in Detoxifying Language Models