Better data centers through machine learning
It’s no secret that we’re obsessed with saving energy. For over a decade we’ve been designing and building data centers that use half the energy of a typical data center, and we’re always looking for ways to reduce our energy use even further. In our pursuit of extreme efficiency, we’ve hit upon a new tool: machine learning. Today we’re releasing a white paper (PDF) on how we’re using neural networks to optimize data center operations and drive our energy use to new lows.
It all started as a 20 percent project, a Google tradition of carving out time for work that falls outside of one’s official job description. Jim Gao, an engineer on our data center team, is well-acquainted with the operational data we gather daily in the course of running our data centers. We calculate PUE, a measure of energy efficiency, every 30 seconds, and we’re constantly tracking things like total IT load (the amount of energy our servers and networking equipment are using at any time), outside air temperature (which affects how our cooling towers work) and the levels at which we set our mechanical and cooling equipment. Being a smart guy—our affectionate nickname for him is “Boy Genius”—Jim realized that we could be doing more with this data. He studied up on machine learning and started building models to predict—and improve—data center performance.
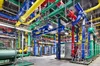
What Jim designed works a lot like other examples of machine learning, like speech recognition: a computer analyzes large amounts of data to recognize patterns and “learn” from them. In a dynamic environment like a data center, it can be difficult for humans to see how all of the variables—IT load, outside air temperature, etc.—interact with each other. One thing computers are good at is seeing the underlying story in the data, so Jim took the information we gather in the course of our daily operations and ran it through a model to help make sense of complex interactions that his team—being mere mortals—may not otherwise have noticed.
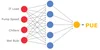
After some trial and error, Jim’s models are now 99.6 percent accurate in predicting PUE. This means he can use the models to come up with new ways to squeeze more efficiency out of our operations. For example, a couple months ago we had to take some servers offline for a few days—which would normally make that data center less energy efficient. But we were able to use Jim’s models to change our cooling setup temporarily—reducing the impact of the change on our PUE for that time period. Small tweaks like this, on an ongoing basis, add up to significant savings in both energy and money.
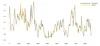
By pushing the boundaries of data center operations, Jim and his team have opened up a new world of opportunities to improve data center performance and reduce energy consumption. He lays out his approach in the white paper, so other data center operators that dabble in machine learning (or who have a resident genius around who wants to figure it out) can give it a try as well.