Why inclusive sets of images help us make better products
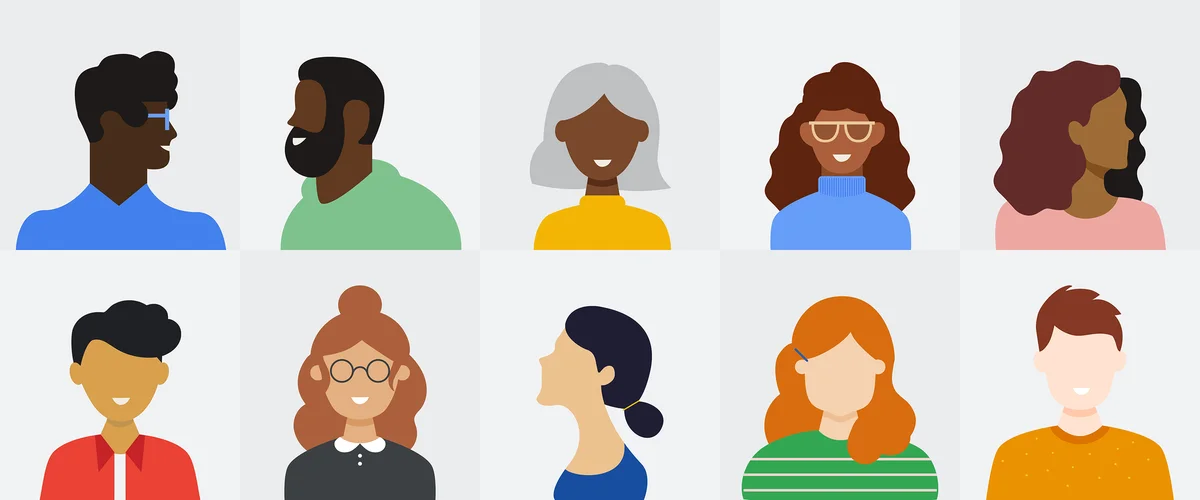
We are committed to making our products more inclusive in a variety of ways. One of the biggest challenges we’ve faced in doing so is finding and using representative data. We want to reflect the experiences and needs of all people who use Google products, particularly people from historically marginalized backgrounds.
When products are not built using diverse and representative data, they can end up being less useful for everyone. So we’ve been retraining some of our earlier machine learning models with more inclusive datasets: sets of data we use to build our hardware and software products.
This is especially important for products that rely on cameras, like taking a photo or using face unlock on your phone. We were able to use more inclusive datasets to create Real Tone on Google Pixel, which represents skin tones authentically and beautifully for all users.
Over the last two years our team partnered with our Responsible Innovation team colleagues to work with the stock photography company TONL, whose name is a nod to the importance of capturing all skin tones accurately and beautifully. They worked with us to source thousands of images of people from historically marginalized backgrounds. We aimed to include photography of models across the gender spectrum, models with darker skin tones, and models with disabilities (and people who represent the intersectionalities of these identities). The project has now expanded to include work with Chronicon and RAMPD to source custom images featuring and centering individuals with chronic conditions and disabilities.
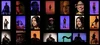
A collection of photos from our work with TONL showcasing people with various skintones, genders, and disabilities.
Google is using these image datasets to help product teams identify potential fairness challenges in the machine learning models they’re developing. We look forward to continuing to improve the representation of our datasets to ensure we’re building the most inclusive and equitable technologies possible for all users.
The Google Skin Tone Team in Responsible AI also worked with TONL to curate the Monk Skin Tone Examples (MST-E) dataset, which includes exemplars of 19 people whose skin tones span the 10-point Monk Skin Tone (MST) scale. The dataset contains 1515 images and 31 videos which captures people in various poses & lighting conditions, as well as with or without accessories like masks or glasses. Because the ways that people classify skin tones can be subjective, Dr. Monk annotated the images of the people featured in the dataset himself. We hope this dataset will help practitioners teach human annotators how to test for consistent skin tone annotations across various conditions, like high and low lighting. Ultimately, this aids in making AI-driven products work better for people of all skin tones.
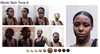
Various images of someone with the Monk Skin Tone 6 classification wearing accessories and in different lighting levels.
Through these projects, we hope to continue working towards our goal to improve skin tone evaluation in machine learning models overall. Visit skintone.google to learn more.