How we built the new family of Gemini Robotics models
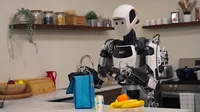
As Google DeepMind prepared for its recent announcement of a new family of Gemini 2.0 models designed specifically for robots, its head of robotics, Carolina Parada, gathered her team for another check of the tech’s capabilities.
They asked a bi-arm ALOHA robot — a duo of limber metal appendages with multiple joints and pincer-like hands used widely in research — to perform tasks it hadn’t done before, using objects it hadn’t seen. “We did random things like put my shoe on the table and ask it to put some pens inside,” Carolina says. “The robot took a moment to understand the task, then did it.”
For the next request, they found a toy basketball hoop and ball and asked the robot to do a “slam dunk.” Carolina watched, proud and delighted, as it did just that.
Carolina says witnessing the slam dunk was a “wow” moment.
“We’d trained models to help robots with specific tasks and to understand natural language before, but this was a step change,” Carolina says. “The robot had never seen anything related to basketball, or this specific toy. Yet it understood something complex — ‘slam dunk the ball’ — and performed the action smoothly. On its first try.”
This all-rounder robot was powered by a Gemini Robotics model that is part of a new family of multimodal models for robotics. The models build upon Gemini 2.0 through fine-tuning with robot-specific data, adding physical action to Gemini’s multimodal outputs like text, video and audio. "This milestone lays the foundation for the next generation of robotics that can be helpful across a range of applications," said Google CEO Sundar Pichai when announcing the new models on X.
The Gemini Robotics models are highly dextrous, interactive and general, meaning they can drive robots to react to new objects, environments and instructions without further training. Helpful, given the team’s ambitions.
“Our mission is to build embodied AI to power robots that help you with everyday tasks in the real world,” says Carolina, whose fascination with robotics began with childhood sci-fi cartoons, fueled by dreams of automated chores. “Eventually, robots will be just another surface on which we interact with AI, like our phones or computers — agents in the physical world.”
Like people, robots need two main functions to perform tasks effectively and safely: the ability to understand and make decisions, and the ability to take action. Gemini Robotics-ER, an "embodied reasoning” model built on Gemini 2.0 Flash, focuses on the former, recognizing elements in front of it, defining their size and location, and predicting the trajectory and grip required to move them. It then can generate code to execute the action. We’re now making this model available to trusted testers and partners.
Google DeepMind is also introducing Gemini Robotics, its most advanced vision-language-action model, which allows robots to reason about a scene, interact with the user and take action. Crucially, it makes significant advances in an area that has proved tricky for roboticists: dexterity. “What comes naturally to humans is difficult for robots,” Carolina explains. “Dexterity requires both spatial reasoning, and complex physical manipulation. Across testing, Gemini Robotics has set a new state-of-the-art for dexterity, solving complex multi-step tasks with smooth motions and great completion times.”
Gemini Robotics-ER excels at embodied reasoning capabilities, including detecting objects and pointing at object parts, finding corresponding points and detecting objects in 3D.
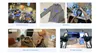
Powered by Gemini Robotics, machines have prepared salads, packed kids’ lunches, played games like Tic-Tac-Toe and even folded an origami fox.
Preparing models that could do many different kinds of tasks was a challenge — largely because it went against the general industry practice of training models for a single task over and over until it can be solved. “Instead, we chose broad task learning, training models on a huge number of tasks,” Carolina says. “We expected to see generalization emerge after a certain amount of time, and we were right.”
Both models can adapt to multiple embodiments, including academic-focused robots, like the bi-arm ALOHA machine, or humanoid robots like Apollo developed by our partner Apptronik.
The models adapt to different embodiments, able to perform tasks like packing a lunchbox or wiping a whiteboard in different forms.
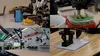
This ability to adapt is key to a future where robots could take on a number of very different roles.
“The possibilities for robots using highly general and capable models are broad and exciting,” Carolina says. “They could be more useful in industries where setups are complex, precision is important and the spaces aren’t human-friendly. And they could be helpful in human-centric spaces, like the home. That’s some years away, but these models are taking us several steps closer.”
Sounds like someone will get some help with those chores — eventually.