How AI can help make safer baby food (and other products)
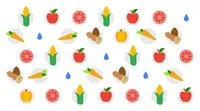
Editor’s note: Whether you’re growing cucumbers or building your own robot arm, machine learning can help. In this guest editorial, Takeshi Ogino of Kewpie tells us how they used machine learning to ensure the quality and safety of the ingredients that go into their food products.
Quality control is a challenge for most industries, but in the world of food production, it’s one of the biggest. With food, products are as good as the ingredients that go into them. Raw materials can vary dramatically, from produce box to produce box, or even from apple to apple. This means inspecting and sorting the good ingredients from the bad is one of the most important tasks any food company does. But all that work inspecting by hand can be time-consuming and arduous both in terms of overhead and manpower. So what’s a food company to do?
At Kewpie Corporation, we turned to a surprising place to explore better ways to ensure food quality: artificial intelligence built on TensorFlow.
Although Kewpie Corporation is most famous for our namesake mayonnaise, we’ve been around for 100 years with dozens of products, from dressings to condiments to baby foods. We’ve always believed that good products begin with good ingredients.
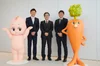
Ingredients that are safe and also give you peace of mind
Last October, we began investigating whether AI and machine learning could ensure the safety and purity of our ingredients faster and more reliably than ever.
The project began with a simple question: “What does it mean to be a ‘good’ ingredient?” The ingredients we purchase must be safe, of course, and from trustworthy producers. But we didn’t think that went far enough. Ingredients also need to offer peace of mind. For example, the color of potatoes can vary in ways that have nothing to do with safety or freshness.
Kewpie depends on manual visual detection and inspection of our raw ingredients. We inspect the entire volume of ingredients used each day, which, at four to five tons, is a considerable workload. The inspection process requires a certain level of mastery, so scaling this process is not easy. At times we’ve been bottlenecked by inspections, and we’ve struggled to boost production when needed.
We’d investigated the potential for mechanizing the process a number of times in the past. However, the standard technology available to us, machine vision, was not practical in terms of precision or cost. Using machine vision meant setting sorting definitions for every ingredient. At the Tosu Plant alone we handle more than 400 types of ingredients, and across the company we handle thousands.
That’s when I began to wonder whether using machine learning might solve our problem.
Using unsupervised machine learning to detect defective ingredients
We researched AI and machine learning technology across dozens of companies, including some dedicated research organizations. In the end, we decided to go with TensorFlow. We were impressed with its capabilities as well as the strength of its ecosystem, which is global and open. Algorithms that are announced in papers get implemented quickly, and there’s a low threshold for trying out new approaches.
One great thing about TensorFlow is that it has such a broad developer community. Through Google, we connected with our development partner, BrainPad Inc, who impressed us with their ability to deliver production level solutions with the latest deep learning. But even BrainPad, who had developed a number of systems to detect defective products in manufacturing processes, had never encountered a company with stricter inspection standards than ours. Furthermore, because our inspections are carried out on conveyor belts, they had to be extremely accurate at high speeds. Achieving that balance between precision and speed was a challenge BrainPad looked forward to tackling.
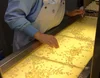
To kick off the project, we started with one of our most difficult inspection targets: diced potatoes. Because they’re an ingredient in baby food, diced potatoes are subject to the strictest scrutiny both in terms of safety and peace of mind. That meant feeding more than 18,000 line photographs into TensorFlow so that the AI could thoroughly learn the threshold between acceptable and defective ingredients.
Our big breakthrough came when we decided to use the AI not as a ”sorter” but an ”anomaly detector.” Designing the AI as a sorter meant supervised learning, a machine learning model that requires labels for each instance in order to accurately train the model. In this case that meant feeding into TensorFlow an enormous volume of data on both acceptable and defective ingredients. But it was hugely challenging for us to collect enough defective sample data. But by training the system to be an anomaly detector we could employ unsupervised learning. That meant we only needed to feed it data on good ingredients. The system was then able to learn how to identify acceptable ingredients, and reject as defective any ingredients that failed to match. With this approach, we achieved both the precision and speed we wanted, with fewer defective samples overall.
By early April, we were able to test a prototype at the Tosu Plant. There, we ran ingredients through the conveyor belt and had the AI identify which ones were defective. We had great results. The AI picked out defective ingredients with near-perfect accuracy, which was hugely exciting to our staff.
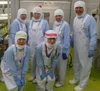
It’s important to note that our goal has always been to use AI to help our plant staff, not replace them. The AI-enabled inspection system performs a rough removal of defective ingredients, then our trained staff inspects that work to ensure nothing slips through. That way we get “good” ingredients faster than ever and are able to process more food and boost production.
Today we may only be working with diced potatoes, but we can’t wait to expand to more ingredients like eggs, grains and so many others. If all goes well, we hope to offer our inspection system to other manufacturers who might benefit. Existing inspection systems such as machine vision have not been universally adopted in our industry because they're expensive and require considerable space. So there’s no question that the need for AI-enabled inspection systems is critical. We hope, through machine learning, we’re bringing even more safe and reassuring products to more people around the world.