MUM: A new AI milestone for understanding information
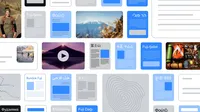
When I tell people I work on Google Search, I’m sometimes asked, "Is there any work left to be done?" The short answer is an emphatic “Yes!” There are countless challenges we're trying to solve so Google Search works better for you. Today, we’re sharing how we're addressing one many of us can identify with: having to type out many queries and perform many searches to get the answer you need.
Take this scenario: You’ve hiked Mt. Adams. Now you want to hike Mt. Fuji next fall, and you want to know what to do differently to prepare. Today, Google could help you with this, but it would take many thoughtfully considered searches — you’d have to search for the elevation of each mountain, the average temperature in the fall, difficulty of the hiking trails, the right gear to use, and more. After a number of searches, you’d eventually be able to get the answer you need.
But if you were talking to a hiking expert; you could ask one question — “what should I do differently to prepare?” You’d get a thoughtful answer that takes into account the nuances of your task at hand and guides you through the many things to consider.
This example is not unique — many of us tackle all sorts of tasks that require multiple steps with Google every day. In fact, we find that people issue eight queries on average for complex tasks like this one.
Today's search engines aren't quite sophisticated enough to answer the way an expert would. But with a new technology called Multitask Unified Model, or MUM, we're getting closer to helping you with these types of complex needs. So in the future, you’ll need fewer searches to get things done.
Helping you when there isn’t a simple answer
MUM has the potential to transform how Google helps you with complex tasks. MUM uses the T5 text-to-text framework and is 1,000 times more powerful than BERT. MUM not only understands language, but also generates it. It’s trained across 75 different languages and many different tasks at once, allowing it to develop a more comprehensive understanding of information and world knowledge than previous models. And MUM is multimodal, so it understands information across text and images and, in the future, can expand to more modalities like video and audio.
Take the question about hiking Mt. Fuji: MUM could understand you’re comparing two mountains, so elevation and trail information may be relevant. It could also understand that, in the context of hiking, to “prepare” could include things like fitness training as well as finding the right gear.
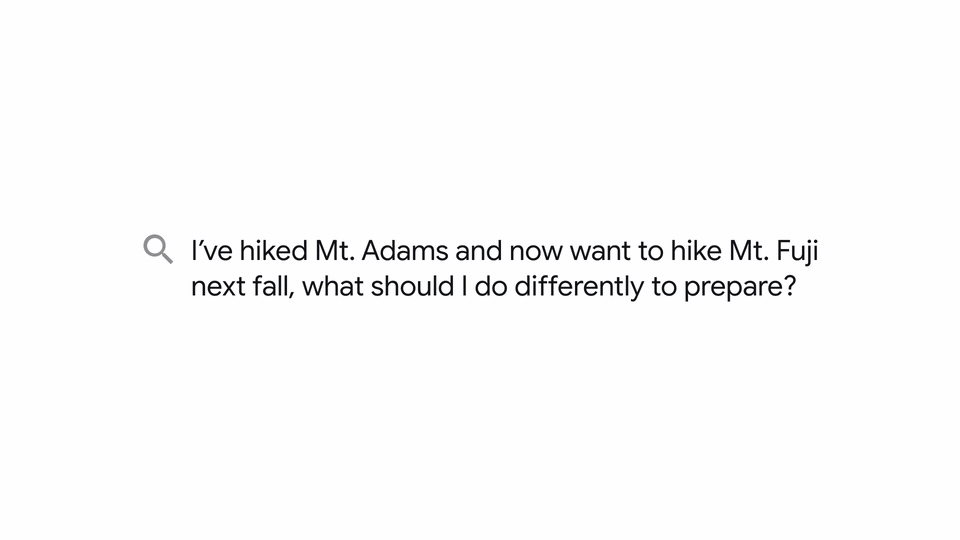
Since MUM can surface insights based on its deep knowledge of the world, it could highlight that while both mountains are roughly the same elevation, fall is the rainy season on Mt. Fuji so you might need a waterproof jacket. MUM could also surface helpful subtopics for deeper exploration — like the top-rated gear or best training exercises — with pointers to helpful articles, videos and images from across the web.
Removing language barriers
Language can be a significant barrier to accessing information. MUM has the potential to break down these boundaries by transferring knowledge across languages. It can learn from sources that aren’t written in the language you wrote your search in, and help bring that information to you.
Say there’s really helpful information about Mt. Fuji written in Japanese; today, you probably won’t find it if you don’t search in Japanese. But MUM could transfer knowledge from sources across languages, and use those insights to find the most relevant results in your preferred language. So in the future, when you’re searching for information about visiting Mt. Fuji, you might see results like where to enjoy the best views of the mountain, onsen in the area and popular souvenir shops — all information more commonly found when searching in Japanese.
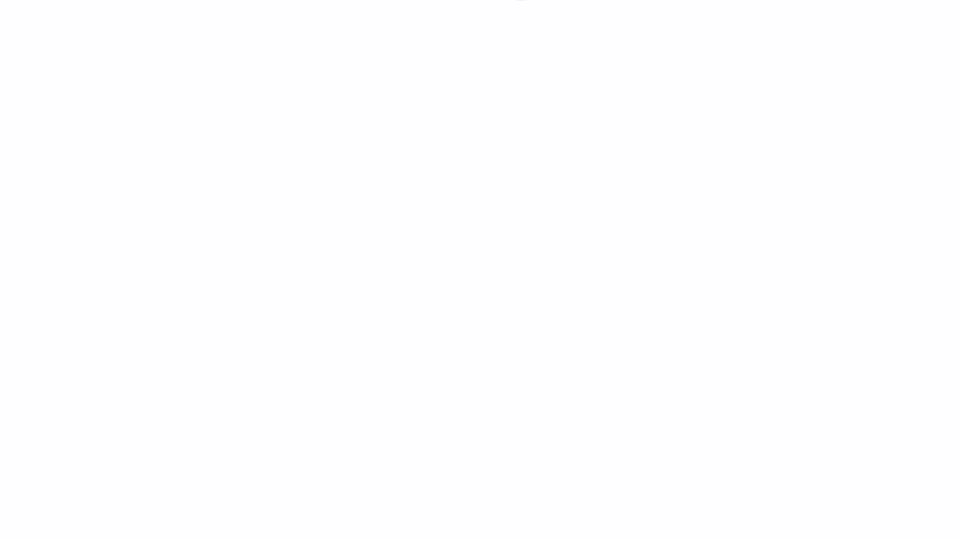
Understanding information across types
MUM is multimodal, which means it can understand information from different formats like webpages, pictures and more, simultaneously. Eventually, you might be able to take a photo of your hiking boots and ask, “can I use these to hike Mt. Fuji?” MUM would understand the image and connect it with your question to let you know your boots would work just fine. It could then point you to a blog with a list of recommended gear.
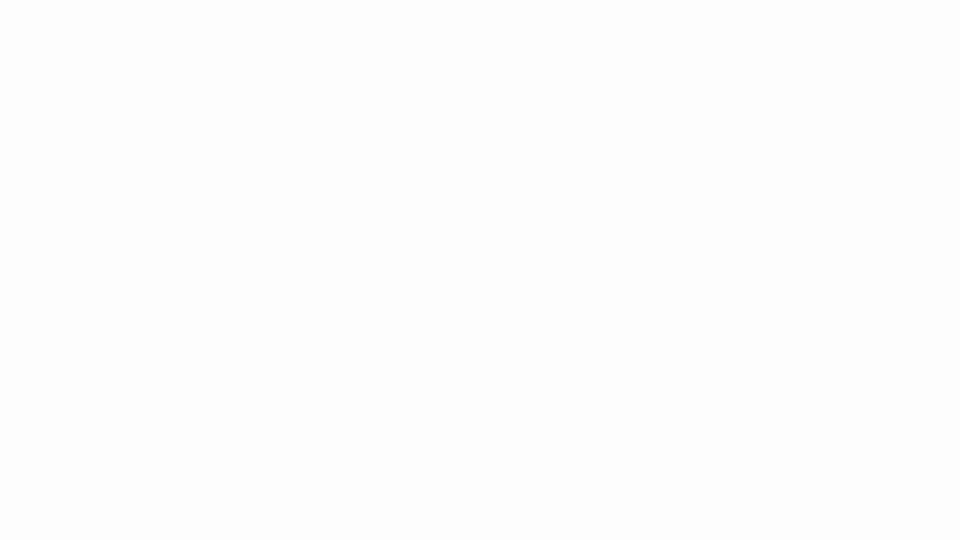
Applying advanced AI to Search, responsibly
Whenever we take a leap forward with AI to make the world’s information more accessible, we do so responsibly. Every improvement to Google Search undergoes a rigorous evaluation process to ensure we’re providing more relevant, helpful results. Human raters, who follow our Search Quality Rater Guidelines, help us understand how well our results help people find information.
Just as we’ve carefully tested the many applications of BERT launched since 2019, MUM will undergo the same process as we apply these models in Search. Specifically, we’ll look for patterns that may indicate bias in machine learning to avoid introducing bias into our systems. We’ll also apply learnings from our latest research on how to reduce the carbon footprint of training systems like MUM, to make sure Search keeps running as efficiently as possible.
We’ll bring MUM-powered features and improvements to our products in the coming months and years. Though we’re in the early days of exploring MUM, it’s an important milestone toward a future where Google can understand all of the different ways people naturally communicate and interpret information.