Fighting fire with machine learning: two students use TensorFlow to predict wildfires
Whenever I get a chance, I pick up my camera and spend hours capturing the immaculate beauty of Big Basin Redwood State Park. It’s been my favorite pastime for years. Giant sequoias, which are the world’s largest single trees and largest living thing by volume, always help me understand our connection with something bigger than ourselves.
Last year, those towering trees were being turned into ashes by wildfires. 2017 was California’s most destructive wildfire season on record, with more than 9,000 fires burning approximately 2,200 square miles of forest. Watching the destruction of centuries-old trees, I challenged myself to find a solution to stop this colossal loss.
When I was 15 years old, I had started a nonprofit organization, Raindrop US, to help raise awareness about the California drought. I also created the “Green Society” at my school, Monta Vista High School, to increase awareness about global warming. It was in meetings of this society last April that I began to partner with Sanjana Shah, a friend and fellow student, to create a permanent solution to wildfire prevention. Sanjana shares my passion for environmental issues and has received multiple national and international awards in the environment space, including the U.S. Presidential Award in 2016.
We decided to develop a device that could identify and predict areas in a forest that are susceptible to wildfires, providing an early warning to fire departments. Available tools measure most of the factors responsible for wildfires like wind speed, wind direction, humidity, temperature. However, biomass, which is created by years of falling branches and trees, is challenging to estimate and measure. Using TensorFlow, Google’s open source machine learning tool, we can analyze images of biomass and estimating their moisture content and size to determine the amount of dead fuel.
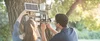
When the moisture content of the downed branches and leaves in the forest is 0 percent, it is categorized as dead fuel. When fuel moisture content is high, fires do not ignite readily, or at all, because most of the fire’s heat energy is used up trying to evaporate and drive water from the plant for it to burn. When the fuel moisture content is low, fires start more easily and can spread rapidly, as all of the heat energy goes directly into the burning flame itself.
Used in a network of sensors, our Smart Wildfire Sensor device could remove the need for fire prevention crews to physically visit forest areas to collect samples of dead fuels and classify them manually. It will also be able to predict the likelihood of wildfire in a forest at a 100 square meter granularity level. Perfect estimation of biomass buildup could significantly impede the speed and ferocity with which these fires spread, reducing costs to fight them and protecting homes and lives.
Last summer, identifying and capturing images of dead fuel accumulated on the forest ground was a significant challenge. However, with the help of Cal Fire in three counties covering forests in the most vulnerable areas for possible wildfires, we were able to capture the necessary images to train the machine learning model. We’re planning to further test our device this summer using ground and aerial drones to capture more images of biomass in wildfire-prone zones to further improve our prediction accuracy.
Just as the giant sequoia trees are undeterred in their striving to reach the heavens, even in destructive wildfires, our resolve to prevent their destruction is equally strong. We’re determined to make full and effective use of high-resolution cameras and available machine learning tools like TensorFlow to precisely predict and prevent irrevocable loss of our most precious nature.