How we built AlphaFold 3 to predict the structure and interaction of all of life’s molecules
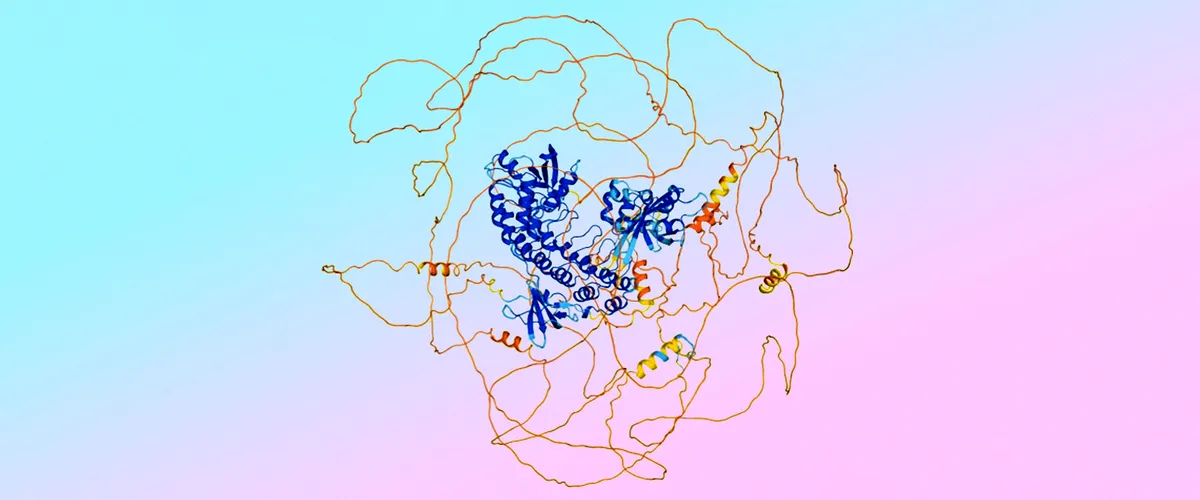
Since its launch in 2020, more than 2 million researchers have used Google DeepMind’s AlphaFold 2 model for protein predictions in their work on vaccine development, cancer treatments and more — helping solve a problem that researchers had been working on for over 50 years. After helping scientists predict hundreds of millions of structures, it would’ve been easy for the team to rest on their laurels.
Instead, they got started on AlphaFold 3. This newer model, which the teams at Google DeepMind and Isomorphic Labs launched in May, builds on our previous models by predicting not just protein folding structures, but predicting the structure and interactions of all of life's molecules, including DNA, RNA and ligands (small molecules that bind to proteins).
“With AlphaFold 2, we made enormous progress on this decades-old open problem of protein folding, but if you look at recent high-impact research, researchers are moving beyond that,” says Jonas Adler, research scientist at Google DeepMind. “Their conclusions were often about something more detailed, like binding of small molecules, or RNA, which AlphaFold 2 couldn’t do. Things had moved on experimentally and so to get to the frontier of where things are today in biology and chemistry, we really needed to be able to cover all biomolecules.”
“Everything” includes ligands, which make up about half of all drugs. “At Isomorphic Labs, we see the tremendous potential of AlphaFold 3 for rational drug design, and we’re already using it in our day-to-day work,” says Adrian Stecula, research leader at Isomorphic Labs. “Investigating the binding of novel small molecules to novel drug targets, answering questions like, ‘How do proteins engage with DNA and RNA?,’ looking into the effects of chemical modifications on protein structure — the new model unlocks all of those capabilities.”
Adding in these additional molecular types introduced an order of magnitude more possible combinations. “Proteins are very ordered. For example, there are only 20 standard amino acids,” Jonas says. “Whereas for small molecules, there's an infinitely large space — they can do basically anything. They're extremely diverse.”
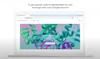
AlphaFold Server allows researchers to access AlphaFold 3.
That meant making a database with all the capabilities would have been impossible. Instead, we’ve released AlphaFold Server, a free tool that lets scientists plug in their own sequences that AlphaFold can then generate molecular complexes for. Since launching in May, researchers have already used it to generate over 1 million structures.
“It’s like Google Maps for molecular complexes,” says Lindsay Willmore, research engineer at Google DeepMind. “Any user who doesn't know how to code at all can just copy and paste the sequences of their proteins, DNA, RNA or the name of their small molecule, press a button and wait a few minutes. Their structure and the confidence metrics will come out so that they're able to look at and evaluate their prediction.”
In order to get AlphaFold 3 to work with this much wider range of biomolecules, the team vastly expanded the data that the newer model was trained on to include DNA, RNA, small molecules and more. “We were able to say, ‘Let's just train on everything that exists in this dataset that helped us so much with proteins and let’s see how far we can get,’” Lindsay says. “And it turns out we can get pretty far.”
Another major change in AlphaFold 3 is a shift in architecture for the final part of the model that generates the structure. Where AlphaFold 2 used a complex custom geometry-based module, AlphaFold 3 uses a generative model that’s based on diffusion — similar to our other cutting-edge image generation models, like Imagen — which greatly simplified how the model handles all the new molecule types.
That shift led to a new issue, though: Since so-called “disordered regions” of proteins weren’t included in the training data, the diffusion model would try to create an inaccurate “ordered” structure with a defined spiral shape, instead of predicting disordered regions.
So the team turned to AlphaFold 2, which is already extremely good at predicting which interactions would be disordered — which look like a pile of chaotic spaghetti — and which ones were not. “We were able to use those predicted structures from AlphaFold 2 as distillation training for AlphaFold 3, so that AlphaFold 3 could learn to predict disorder,” Lindsay says.
“We have a saying: ‘Trust the fusilli, reject the spaghetti,’” adds Jonas.
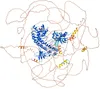
An example of a prediction from AlphaFold 3 with ordered “fusilli” regions in blue and disordered “spaghetti” regions in orange. The colors represent the model’s confidence of predicted accuracy.
The team is looking forward to seeing how researchers will use AlphaFold 3 to advance fields like genomics research, drug design and more.
“It's incredible to see how much progress we made,” Jonas says. “What used to be very hard has become very easy. What used to be impossible has become possible — and while there are still very hard problems here to solve, we’re excited about the potential for AlphaFold 3 to help solve them.”