Machine learning gives environmentalists something to tweet about
Editor’s note: TensorFlow, our open source machine learning library, is just that—open to anyone. Companies, nonprofits, researchers and developers have used TensorFlow in some pretty cool ways, and we’re sharing those stories here on Keyword. Here’s one of them.
Victor Anton captured tens of thousands of birdsong recordings, collected over a three-year period. But he had no way to figure out which birdsong belonged to what bird.
The recordings, taken at 50 locations around a bird sanctuary in New Zealand known as “Zealandia,” were part of an effort to better understand the movement and numbers of threatened species including the Hihi, Tīeke and Kākāriki. Because researchers didn’t have reliable information about where the birds were and how they moved about, it was difficult to make good decisions about where to target conservation efforts on the ground.
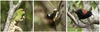
That’s where the recordings come in. Yet the amount of audio data was overwhelming. So Victor—a Ph.D. student at Victoria University of Wellington, New Zealand—and his team turned to technology.
“We knew we had lots of incredibly valuable data tied up in the recordings, but we simply didn’t have the manpower or a viable solution that would help us unlock this,” Victor tells us. “So we turned to machine learning to help us.”
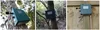
In one of the quirkier applications of machine learning, they trained a Google TensorFlow-based system to recognize specific bird calls and measure bird activity. The more audio it deciphered, the more it learned, and the more accurate it became.
It worked like this: the AI system used audio that had been recorded and stored, chopping it into minute-long segments, and then converting the file into a spectrogram. After the spectrograms were chopped into chunks, each spanning less than a second, they were processed individually by a deep convolutional neural network. A recurrent neural network then tied together the chunks and produced a continual prediction of which of the three birds was present across the minute-long segment. These segments were compiled to create a fuller picture about the presence and movement of the birds.
TensorFlow processed the Spectograms and learned to identify the calls of different species.
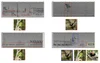
The team faced some unique challenges. They were starting with a small quantity of labelled data, the software would often pick up other noises like construction, cars and even doorbells, and some of the bird species had a variety of birdsongs or two would sing at the same time.
To overcome these hurdles, they tested, verified and retrained the system many times over. As a result, they have learned things that would have otherwise remained locked up in thousands of hours of data. While it’s still early days, already conservation groups are talking to Victor about how they can use these initial results to better target their efforts. Moreover, the team has seen enough encouraging signs that they believe that their tools can be applied to other conservation projects.
“We are only just beginning to understand the different ways we can put machine learning to work in helping us protect different fauna,” says Victor, “ultimately allowing us to solve other environmental challenges across the world.”