5 myths about medical AI, debunked
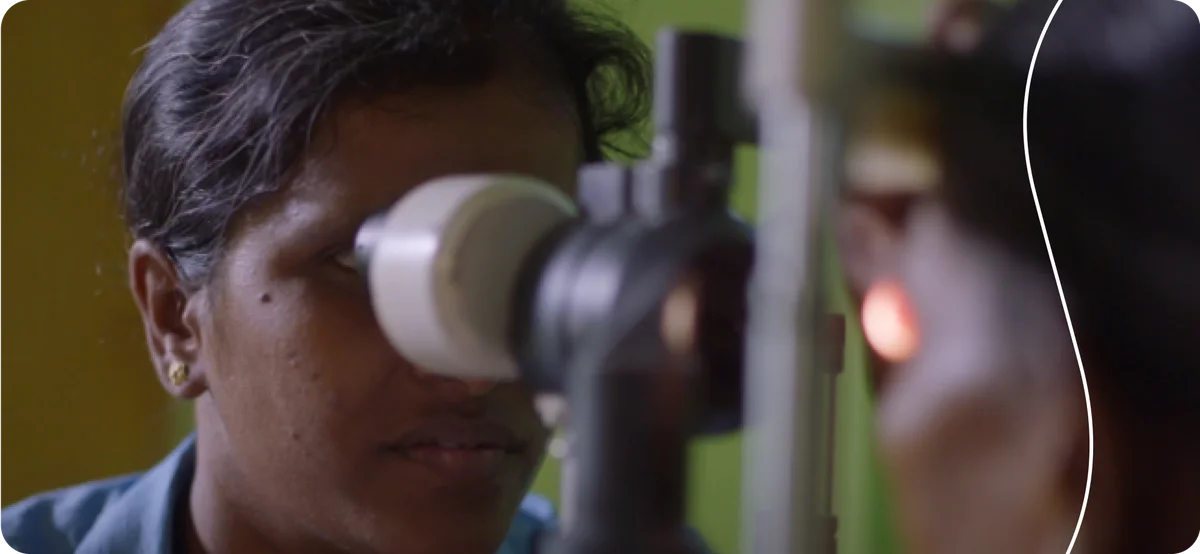
In 2015, a small research team at Google brainstormed how AI could help improve people’s health. We met doctors like Dr. Kim Ramasamy at Aravind Eye Hospital, who had a lifelong vision of improving access to eyesight-saving patient care. There are not enough specialists in many parts of the world, and having an AI-powered screening system could help doctors reach more patients. We set out to explore whether we could train AI to identify diabetic retinopathy (DR), a leading and growing cause of preventable blindness, and in 2016, we built an AI model that performed on par with eyecare doctors.
In 2018, our partner team at Verily received CE mark for this tool, Automated Retinal Disease Assessment (ARDA). As the prevalence of diabetes rises in low- and middle-income countries, we felt it was most critical to assist with the rising demand and the first patient was screened with ARDA in Madurai, India.
Today, ARDA has screened over 200,000 patients in clinics around the world, from urban cities in the EU to rural communities in India. However, the path to bringing medical AI into a real clinical environment was not easy. To help others who may be embarking on a similar journey, we are sharing our key lessons learned in an article published in Nature Medicine.
Below are 5 key lessons we learned from our work:
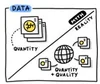
Myth: The more data, the better
Reality: While data volume is important in developing an accurate AI model, data quality matters more. Training data should represent data diversity in the real world (e.g., patient demography, data quality reflecting real conditions, etc.) and having experts adjudicate tough cases will improve labeling quality.
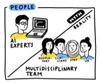
Myth: AI experts are all you need
Reality: Building a well-functioning medical AI system takes a village of multidisciplinary teams including clinicians, designers, human computer interaction researchers, regulatory, ethical, legal experts, and more.
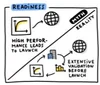
Myth: High AI performance equals clinical confidence
Reality: Validating the performance of AI in a controlled setting does not guarantee the same level of performance when it’s rolled out to real clinics. Careful validations in real-world environments are necessary to ensure AI’s robust performance and model generalizability.
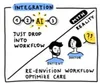
Myth: It’s easy to fit AI into existing workflows
Reality: We need to design AI around humans, not the other way around. Sometimes the best AI use cases may be different than the original assumptions. We’ve also observed that adding AI into a workflow sometimes prompted unanticipated adjustments in the overall clinical processes, such as optimization in patient education and patient scheduling
The prior expectations (“myths”) and learnings (“reality”) of developing and deploying medical AI
[Image source: Anna Iurchenko]
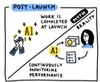
Myth: Launch means success
Reality: Patient population or environmental factors may change after the initial launch. These factors can affect AI’s performance unexpectedly. Implementing a system to proactively monitor AI’s performance can help detect potential issues early.
Research in medical AI model development has increased exponentially in recent years. However, research on how to deploy medical AI is often overlooked. While many aspects, such as regulations for medical AI deployment and monitoring are still evolving in many parts of the world, we hope these learnings can help to facilitate discussions around ensuring that medical AI is deployed safely and effectively to benefit patients.