Mahima Pushkarna is making data easier to understand
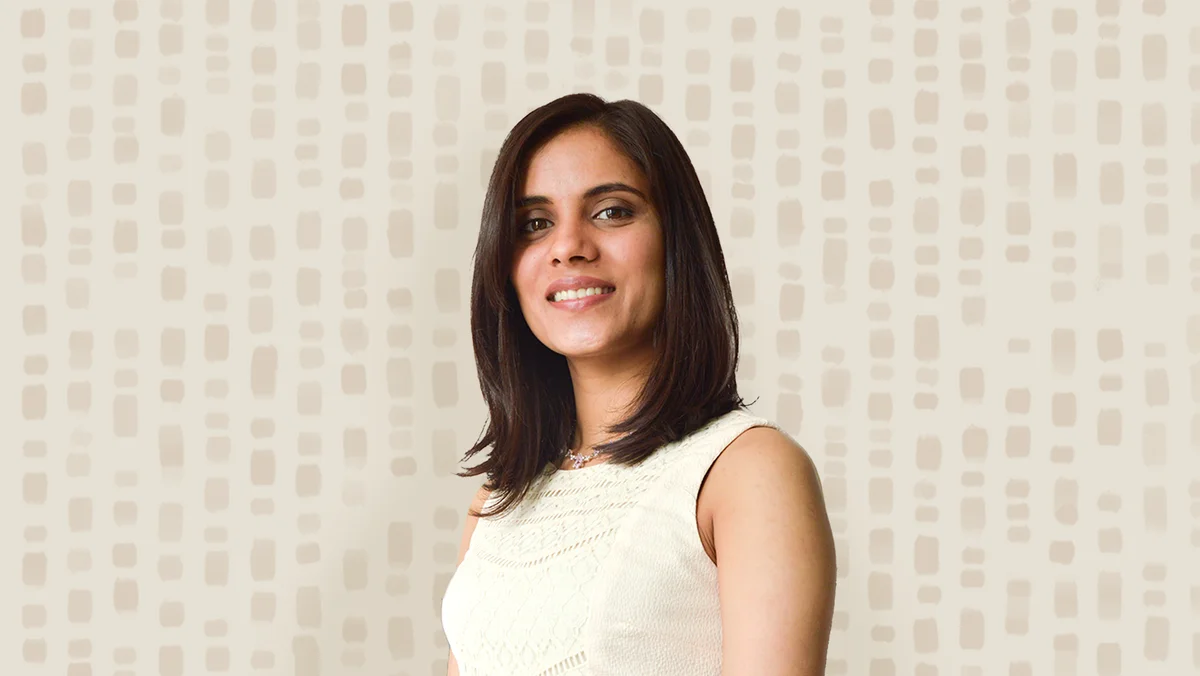
Five years ago, information designer Mahima Pushkarna joined Google to make data easier to understand. As a senior interaction designer on the People + AI Research (PAIR) team, she designed Data Cards to help everyone better understand the contexts of the data they are using. The Data Cards Playbook puts Google’s AI Principles into practice by providing opportunities for feedback, relevant explanations and appeal.
Recently, Mahima’s paper on Data Cards (co-written with Googlers Andrew Zaldivar and Oddur Kjartansson) was accepted to the ACM Conference on Fairness, Accountability and Transparency (ACM FAccT). Let’s catch up with her and find out more about what brought her to Google.
How did your background lead you to the work you’re doing now?
I've always been fascinated by conjuring up solutions to things. The kind of questions that I’ve found meaningful are those that are never truly solved, or never have one correct answer. (The kind of questions that exasperate us!) Those have been the problems I am always drawn towards.
Early in my career, I realized the power in visualizing data, but spreadsheets were intimidating. I wondered how design could make communicating complexity easier. So I found myself in grad school in Boston studying information design and data visualization. I focused on how people experience data and how our relationships to each other and our contexts are mediated.
I joined Google Brain as the first visual designer in a full-time capacity, though I had no background in artificial intelligence or machine learning — this was the deep end of the pool. This opened up the space to explore human-AI interaction, and make AI more accessible to a broader class of developers. At PAIR, my work focuses on making information experiences more meaningful for developers, researchers and others who build AI technologies.
What’s it like to have a unique background as a designer on a technical AI research team?
When you're an engineer and immersed in building technology, it's easy to assume everyone has a similar experience to your own — especially when you’re surrounded by peers who share your expertise. The actual user experience is very personal and varies drastically across users and contexts. That particular clarity is what designers bring to the table.
I’ve been able to engage my engineering and research colleagues with simple, people-centered questions right in the very beginning. How are people using an AI tool? What are they learning from it? Who else might be involved in the conversation? Do they have the proficiency we assume they have?
How did you begin designing Data Cards?
This project started when I was working on another visualization toolkit, Facets, to communicate the skews and imbalances within datasets to help machine learning practitioners make informed decisions. At the time, transparency was a moving target. Andrew, Tulsee Doshi and I started to proactively think about fairness in data, and saw a huge gap in the documentation of human decisions that dot a dataset's lifecycle.
This “invisible” information shapes how we use data and the outcomes of models trained on them. For example, a model trained on a dataset that captures age in just two or three buckets will have very different outcomes compared to a dataset with ten buckets. The goal of Data Cards is to make both visible and invisible information about datasets available and simple to understand, so people from a variety of backgrounds can knowledgeably make decisions.
As we cover in our FAccT paper, Andrew and Oddur and I arrived at two insights. The first is that identifying what we don’t know about data is just as important as articulating what we do know. In capturing these nuances, it is possible to narrow those knowledge gaps before even collecting data. The second thing that surprised us was the sheer number of people involved in a dataset’s life cycle, and how fragile knowledge is. Context is easily lost in translation both between and within teams, across documents, emails, people and time.
Data Cards stand on the shoulders of giants, like Data Sheets (Gebru, et al.) and Model Cards (Mitchell et al.). We've been immensely lucky to have had the support of many original authors on these seminal papers that have paved our path to FAccT.
Identifying what we don’t know about data is just as important as articulating what we do know.
How do you hope the paper is used across the tech industry?
Imagine a world in which finding verifiable information about the motivations of a dataset’s creators or performance of a model is as easy as learning about the ethical beliefs of a celebrity or the rating of a movie. Our vision for Data Cards is that they become a cultural mainstay — invisible, but their absence would be missed by ML practitioners.
In this paper, we introduce frameworks that other teams can use in their work. Alongside that, we’ve open-sourced the Data Cards Playbook, so we're trying to lower the barrier to access in every way possible.