PAIR: the People + AI Research Initiative
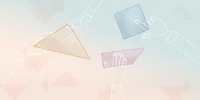
The past few years have seen rapid advances in machine learning, with dramatic improvements in technical performance—from more accurate speech recognition, to better image search, to improved translations. But we believe AI can go much further—and be more useful to all of us—if we build systems with people in mind at the start of the process.
Today we’re announcing the People + AI Research initiative (PAIR) which brings together researchers across Google to study and redesign the ways people interact with AI systems. The goal of PAIR is to focus on the "human side" of AI: the relationship between users and technology, the new applications it enables, and how to make it broadly inclusive. The goal isn’t just to publish research; we’re also releasing open source tools for researchers and other experts to use.
PAIR's research is divided into three areas, based on different user needs:
Engineers and researchers: AI is built by people. How might we make it easier for engineers to build and understand machine learning systems? What educational materials and practical tools do they need?
Domain experts: How can AI aid and augment professionals in their work? How might we support doctors, technicians, designers, farmers, and musicians as they increasingly use AI?
Everyday users: How might we ensure machine learning is inclusive, so everyone can benefit from breakthroughs in AI? Can design thinking open up entirely new AI applications? Can we democratize the technology behind AI?
We don't have all the answers—that's what makes this interesting research—but we have some ideas about where to look. One key to the puzzle is design thinking. Instead of viewing AI purely as a technology, what if we imagine it as a material to design with? Here history might serve as a guide: For instance, advances in computer graphics meant more than better ways of drawing pictures—and that led to completely new kinds of interfaces and applications. You can read more in this post on what we call human-centered machine learning (HCML).We’re open sourcing new tools, creating educational materials (such as guidelines for designing AI interfaces), and publishing research to answer these questions and spread the power of AI to as many people as possible.
Open-source tools
Today we're open sourcing two visualization tools, Facets Overview and Facets Dive. These applications are aimed at AI engineers, and address the very beginning of the machine learning process. The Facets applications give engineers a clear view of the data they use to train AI systems.
We think this is important because training data is a key ingredient in modern AI systems, but it can often be a source of opacity and confusion. Indeed, one of the ways that ML engineering seems different than traditional software engineering is a stronger need to debug not just code, but data too. With Facets, engineers can more easily debug and understand what they’re building. You can read full details at our open source repository.
Supporting external research
We also acknowledge that we're not the first to see this opportunity or ask these questions. Many designers and academics have started exploring human/AI interaction. Their work inspires us; we see community-building and research support as an essential part of our mission. We’re working with a pair of visiting academics—Prof. Brendan Meade of Harvard and Prof. Hal Abelson of MIT—who are focusing on education and science in the age of AI.
Focusing on the human element in AI brings new possibilities into view. We're excited to work together to invent and explore what's possible.