Partnering on machine learning in healthcare
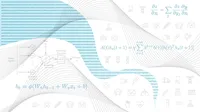
From 1816’s stethoscope to today’s handheld ultrasound machines, doctors have steadily adopted technology to advance healthcare. In the past decade, the medical community has taken a major step in adopting electronic health systems to make information more accessible to clinicians and patients. Now, to make sense of all that information, healthcare providers are starting to turn to machine learning.
Our researchers at Google have shown over the past year how our machine learning can help clinicians detect breast cancer metastases in lymph nodes and screen for diabetic retinopathy. We’re working with Alphabet’s Verily arm and other biomedical partners to translate these research results into practical medical devices, such as a tool to help prevent blindness in patients with diabetes.
Now we’re ready to do more: machine learning is mature enough to start accurately predicting medical events—such as whether patients will be hospitalized, how long they will stay, and whether their health is deteriorating despite treatment for conditions such as urinary tract infections, pneumonia, or heart failure. Advanced machine learning can discover patterns in de-identified medical records (that is, stripped of any personally identifiable information) to predict what is likely to happen next, and thus, anticipate the needs of the patients before they arise. So we’ve partnered with world-class medical researchers and bioinformaticians at UC San Francisco, Stanford Medicine and University of Chicago Medicine to explore how machine learning combined with clinical expertise could improve patient outcomes, avoid costly incidents and save lives.
The need is urgent and immense: each year in the U.S., healthcare-associated infections lead to 99,000 deaths, problems with medications cause more than 770,000 injuries and deaths and unplanned hospital readmissions cost as much as $17 billion. There are also pressing global issues: every year 43 million people worldwide are affected by medical errors, most in middle- to low-income countries. While significant headway has already been made, a number of clinicians have reached out to Google to ask whether better use of health information could produce better outcomes.
Healthcare data is very complex, and we spent this past year validating the data and developing the techniques. Our results will be undergoing clinical review over the next few months to ensure the approach is rigorous.
We’re also helping to harmonize the different ways data appears across organizations. Each clinic has its own way of recording data, which makes gathering insights from multiple clinics difficult. And the lack of data interoperability and standardization makes resolving these differences a time-consuming manual process. But now, using our deep learning technology on top of the open data standard FHIR, we can automate parts of this process, making it easier for clinicians and researchers to use the data.
As part of this research, our healthcare partners ensured that patient data was appropriately de-identified prior to sharing. We then used Google Cloud’s infrastructure to keep the data stored securely with the highest level of protections and to strictly follow HIPAA privacy rules. The records are kept separate from consumer data and will only be used in our partnership research projects.
We believe clinical breakthroughs using machine learning will come only when the medical community and deep learning experts collaborate closely. Most of us at Google are not doctors, but everyone has been touched by an illness or injury, or even lost a loved one. We have something unique at Google that we can contribute to make care better—so we must try. We look forward to growing our healthcare partnerships in the hopes that together, we can improve the health of many millions of people worldwide.
Note: We’re always open to collaborations on large medical datasets of all types. If you know of an organization that would like to work with us, please fill out this this form.