An update on our progress in responsible AI innovation
Over the past year, responsibly developed AI has transformed health screenings, supported fact-checking to battle misinformation and save lives, predicted Covid-19 cases to support public health, and protected wildlife after bushfires. Developing AI in a way that gets it right for everyone requires openness, transparency, and a clear focus on understanding the societal implications. That is why we were among the first companies to develop and publish AI Principles and why, each year, we share updates on our progress.
Internal Education
In the last year, to ensure our teams have clarity from day one, we’ve added an introduction to our AI Principles for engineers and incoming hires in technical roles. The course presents each of the Principles as well as the applications we will not pursue.
Integrating our Principles into the work we do with enterprise customers is key, so we’ve continued to make our AI Principles in Practice training mandatory for customer-facing Cloud employees. A version of this training is available to all Googlers.
There is no single way to apply the AI Principles to specific features and product development. Training must consider not only the technology and data, but also where and how AI is used. To offer a more comprehensive approach to implementing the AI Principles, we’ve been developing opportunities for Googlers to share their points of view on the responsible development of future technologies, such as the AI Principles Ethics Fellowship for Google’s Employee Resource Groups. Fellows receive AI Principles training and craft hypothetical case studies to inform how Google prioritizes socially beneficial applications. This inaugural year, 27 fellows selected from 191 applicants from around the world wrote and presented case studies on topics such as genome datasets and a Covid-19 content moderation workflow.
Other programs include a bi-weekly Responsible AI Tech Talk Series featuring external experts, such as the Brookings Institution’s Dr. Nicol Turner Lee presenting on detecting and mitigating algorithmic bias.
Tools and Research
To bring together multiple teams working on technical tools and research, this year we formed the Responsible AI and Human-Centered Technology organization. The basic and applied researchers in the organization are devoted to developing technology and best practices for the technical realization of the AI Principles guidance.
As discussed in our December 2020 End-of-Year report, we regularly release these tools to the public. Currently, researchers are developing Know Your Data (in beta) to help developers understand datasets with the goal of improving data quality, helping to mitigate fairness and bias issues.
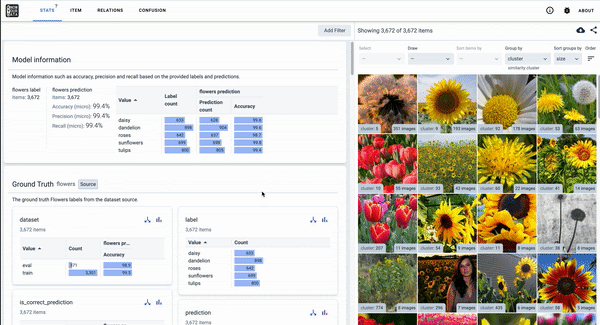
Know Your Data, a Responsible AI tool in beta
Product teams use these tools to evaluate their work’s alignment with the AI Principles. For example, the Portrait Light feature available in both Pixel’s Camera and Google Photos uses multiple machine learning components to instantly add realistic synthetic lighting to portraits. Using computational methods to achieve this effect, however, raised several responsible innovation challenges, including potentially reinforcing unfair bias (AI Principle #2) despite the goal of building a feature that works for all users. So the Portrait Light team generated a training dataset containing millions of photos based on an original set of photos of different people in a diversity of lighting environments, with their explicit consent. The engineering team used various Google Responsible AI tools to test proactively whether the ML models used in Portrait Light performed equitably across different audiences.
Our ongoing technical research related to responsible innovation in AI in the last 12 months has led to more than 200 research papers and articles that address AI Principles-related topics. These include exploring and mitigating data cascades; creating the first model-agnostic framework for partially local federated learning suitable for training and inference at scale; and analyzing the energy- and carbon-costs of training six recent ML models to reduce the carbon footprint of training an ML system by up to 99.9%.
Operationalizing the Principles
To help our teams put the AI Principles into practice, we deploy our decision-making process for reviewing new custom AI projects in development — from chatbots to newer fields such as affective technologies. These reviews are led by a multidisciplinary Responsible Innovation team, which draws on expertise in disciplines including trust and safety, human rights, public policy, law, sustainability, and product management. The team also advises product areas, on specific issues related to serving enterprise customers. Any Googler, at any level, is encouraged to apply for an AI Principles review of a project or planned product or service.
Teams can also request other Responsible Innovation services, such as informal consultations or product fairness testing with the Product Fairness (ProFair) team. ProFair tests products from the user perspective to investigate known issues and find new ones, similar to how an academic researcher would go about identifying fairness issues.
Our Google Cloud, Image Search, Next Billion Users and YouTube product teams have engaged with ProFair to test potential new projects for fairness. Consultations include collaborative testing scenarios, focus groups, and adversarial testing of ML models to address improving equity in data labels, fairness in language models, and bias in predictive text, among other issues. Recently, the ProFair team spent nine months consulting with Google researchers on creating an object recognition dataset for physical landmarks (such as buildings), developing criteria for how to choose which classes to recognize and how to determine the amount of training data per class in ways that would assign a fairer relevance score to each class.
Reviewers weigh the nature of the social benefit involved and whether it substantially exceeds potential challenges. For example, in the past year, reviewers decided not to publicly release ML models that can create photo-realistic synthetic faces made with generative adversarial networks (GANs), because of the risk of potential misuse by malicious actors to create “deepfakes” for misinformation purposes.
As another example, a research team requested a review of a new ML training dataset for computer vision fairness techniques that offered more specific attributes about people, such as perceived gender and age-range. The team worked with Open Images, an open data project containing ~9 million images spanning thousands of object categories and bounding box annotations for 600 classes. Reviewers weighed the risk of labeling the data with the sensitive labels of perceived gender presentation and age-range, and the societal benefit of these labels for fairness analysis and bias mitigation. Given these risks, reviewers required creation of a data card explaining the details and limitations of the project. We released the MIAP (More Inclusive Annotations for People) dataset in the Open Images Extended collection. The collection contains more complete bounding box annotations for the person class hierarchy in 100K images containing people. Each annotation is also labeled with fairness-related attributes. MIAP was accepted and presented at the 2021 Artificial Intelligence, Ethics and Society conference.
External Engagement
We remain committed to contributing to emerging international principles for responsible AI innovation. For example, we submitted a response to the European Commission consultation on the inception impact assessment on ethical and legal requirements for AI and feedback on NITI Aayog’s working document on Responsible Use of AI to guide national principles for India. We also supported the Singaporean government’s Guide to Job Redesign in the Age of AI, which outlines opportunities to optimize AI benefits by redesigning jobs and reskilling employees.
Our efforts to engage global audiences, from students to policymakers, center on educational programs and discussions to offer helpful and practical ML education, including:
- A workshop on Federated Learning and Analytics, making all research talks and a TensorFlow Federated tutorial publicly available.
- Machine Learning for Policy Leaders (ML4PL), a 2-hour virtual workshop on the basics of ML. To date, we’ve expanded this globally, reaching more than 350 policymakers across the EU, LatAm, APAC, and US.
- A workshop co-hosted with the Indian Ministry of Electronics and Information Technology on the Responsible Use of AI for Social Empowerment, exploring the potential of AI adoption in the government to address COVID-19, agricultural and environmental crises.
To support these workshops and events with actionable and equitable programming designed for long-term collaboration, over the past year we’ve helped launch:
- AI for Social Good workshops, bringing together NGOs applying AI to tough challenges in their communities with academic experts and Google researchers to encourage collaborative AI solutions. So far we’ve supported more than 30 projects in Asia Pacific and Sub-Saharan Africa with expertise, funding and Cloud Credits.
- Two collaborations with the U.S. National Science Foundation: one to support the National AI Research Institute for Human-AI Interaction and Collaboration with $5 million in funding, along with AI expertise, research collaborations and Cloud support; another to join other industry partners and federal agencies as part of a combined $40 million investment in academic research for Resilient and Intelligent Next-Generation (NextG) Systems, in which Google will offer expertise, research collaborations, infrastructure and in-kind support to researchers.
- Quarterly Equitable AI Research Roundtables (EARR), focused on the potential downstream harms of AI with experts from the Othering and Belonging Institute at UC Berkeley, PolicyLink, and Emory University School of Law.
- MLCommons, a non-profit, which will administer MLPerf, a suite of benchmarks for Google and industry peers.
Committed to sharing tools and discoveries
In the three years since Google released our AI Principles, we’ve worked to integrate this ethical charter across our work — from the development of advanced technologies to business processes. As we learn and engage with people and organizations across society we’re committed to sharing tools, processes and discoveries with the global community. You’ll find many of these in our recently updated People + AI Research Guidebook and on the Google AI responsibilities site, which we update quarterly with case studies and other resources.