A shared agenda for responsible AI progress
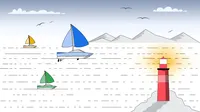
Generative AI experiments like Bard, tools like the PaLM and MakerSuite APIs and a growing number of services from across the AI ecosystem have sparked excitement about AI’s transformative potential — and concern about potential misuse.
It’s helpful to recall that AI is simultaneously revolutionary and something that’s been quietly helping us out for years.
If you’ve searched the internet or used a modern smartphone, you were working with AI — you just may not have noticed it making things better (and safer) behind the scenes. (Here’s a deeper dive on why we’ve been focused on AI.)
AI has been powering Google’s core products for years. Learn more in this article.
Search: AI lets you search in a new language, search with your camera, search by humming a tune, even search with images and text at the same time.
Maps: The AI behind Google Maps gives you up-to-date traffic information, suggests eco-friendly routes and helps you avoid traffic jams.
Photos: Back in 2015 we developed AI in Google Photos to help you search for and sort your photos based on who’s pictured.
Assistant: The Natural Language Processing AI technology developed for Assistant allows your phone, your Home, your TV or your car to parse the meaning of your questions and respond appropriately.
Gmail: AI powers our autocomplete and spell check are powered by AI, as is our spam filtering, which prevents more than 99.9% of spam, phishing attempts and malware from reaching you.
Cloud: Google Cloud has built AI into countless solutions that our customers can customize for document processing, call center operations, video and image analysis and translation.
But AI is now opening new possibilities in scientific fields as varied as personalized medicine, precision agriculture, nuclear fusion, water desalination and materials science — what DeepMind co-founder Demis Hassabis has called “scientific exploration at digital speed.” The technology’s incredible ability to predict everything from future disasters to protein structures is taking center stage. And it may be a critical part of humanity’s ability to address global challenges ranging from climate change to food scarcity.
What will it take to realize this potential? My colleague James Manyika poses a thought experiment:
It’s the year 2050. AI has turned out to be hugely beneficial to society. What happened? What opportunities did we realize? What problems did we solve?
The most obvious and basic answer, and the starting point for deeper conversations, is that we would have needed citizens, educators, academics, civil society and governments to come together to help shape the development and use of AI.
What does responsible AI mean?
There's been much discussion about AI responsibility across the industry and civil society, but a widely accepted definition has remained elusive. AI responsibility has come to be associated with just avoiding risks, but we actually see it as having two aspects: Not just mitigating complexities and risks, but also helping improve people's lives and addressing social and scientific challenges. Some elements — like the need to incorporate important social values like accuracy, privacy, fairness, transparency and more — are widely agreed, although striking the right balances between them can be complex. Others — like how quickly and broadly to deploy the latest advances — are more controversial.
In 2018, we were one of the first companies to publish a set of AI Principles. Learning from the experience of the internet, with all of its benefits and challenges, we’ve tried to build smart checks and balances into our products, designing for social benefit from the ground up.
Our first AI Principle — that AI should “be socially beneficial” — captures the critical balance at stake: “The overall likely benefits should substantially exceed the foreseeable risks and downsides.”
And what does it take?
Putting that aspiration into practice is demanding, and it’s work we’ve been doing for years. We started working on Machine Learning Fairness back in 2014, three years before we published our groundbreaking research on Transformer — the foundation for modern language models.
Since then, we’ve continued evolving our practices, conducting industry-leading research on AI impacts and risk management, assessing proposals for new AI research and applications to ensure they align with our principles and publishing nearly 200 papers to support and develop industry norms. And we’re constantly reassessing the best ways to build accountability and safety into our work, and publishing our progress.
A few examples: In 2021 DeepMind published an early paper on the ethical issues associated with Large Language Models (LLMs). In 2022 we shared innovations in Audio Machine Learning (and the capacity to detect synthetic speech). That same year we released the Monk Skin Tone Scale and began incorporating it into products like Image Search, Photos and Pixel, more accurately capturing a range of skin tones. And in 2022, as part of our publication of our PaLM model, we shared learnings on pre-assessments of the outputs of LLMs.
With input from a broad spectrum of voices, we weigh both the benefits and risks of launching — and not launching — products. And we've been deliberately restrained in deploying some AI tools.
For example, in 2018 we announced we were not releasing a general-purpose API for facial recognition, even as others plowed ahead before there was a legal or technical framework for acceptable use. And when one of our teams developed an AI model for lip-reading that could help people with hearing or speaking impairments, we ran a rigorous review to make sure it would not be useful for mass surveillance before publishing a research paper.
In rolling out Bard to a growing number of users, we prioritized feedback, safety and accountability, building in guardrails like caps on the number of exchanges in a dialogue to ensure interactions remained helpful and on topic, and carefully setting expectations for what the model could and couldn’t accomplish. As American philosopher Daniel Dennett has observed, it’s important to distinguish competence from comprehension, and we realize that we’re early on this journey. In the coming weeks and months, we’ll continue improving on this generative AI experiment based on feedback.
We recognize that cutting-edge AI developments are emergent technologies — that learning how to assess their risks and capabilities goes well beyond mechanically programming rules into the realm of training models and assessing outcomes.
How can we work better together?
No one company can progress this approach alone. AI responsibility is a collective-action problem — a collaborative exercise that requires bringing multiple perspectives to the table to help get to the right balances. What Thomas Friedman has called “complex adaptive coalitions.” As we saw with the birth of the internet, we all benefit from shared standards, protocols and institutions of governance.
That’s why we’re committed to working in partnership with others to get AI right. Over the years we’ve built communities of researchers and academics dedicated to creating standards and guidance for responsible AI development. We collaborate with university researchers at institutions like the Australian National University, the University of California Berkeley, the Data & Society Research Institute, the University of Edinburgh, McGill, the University of Michigan, the Naval Postgraduate School, Stanford and the Swiss Federal Institute of Technology.
And to support international standards and shared best practices, we’ve contributed to ISO and IEC Joint Technical Committee’s standardization program in the area of Artificial Intelligence.
These partnerships offer a chance to engage with multi-disciplinary experts on complex AI research questions related to AI — some scientific, some ethical, some cutting across domains.
What will it take to get AI public policy right?
When it comes to AI, we need both good individual practices and shared industry standards. But society needs something more: Sound government policies that promote progress while reducing risks of abuse. And developing good policy takes deep discussions across governments, the private sector, academia and civil society.
As we’ve said for years, AI is too important not to regulate — and too important not to regulate well. The challenge is to do it in a way that mitigates risks and promotes trustworthy applications that live up to AI’s promise of societal benefit.
Here are some core principles that can help guide this work:
- Build on existing regulation, recognizing that many regulations that apply to privacy, safety or other public purposes already apply fully to AI applications.
- Adopt a proportionate, risk-based framework focused on applications, recognizing that AI is a multi-purpose technology that calls for customized approaches and differentiated accountability among developers, deployers and users.
- Promote an interoperable approach to AI standards and governance, recognizing the need for international alignment.
- Ensure parity in expectations between non-AI and AI systems, recognizing that even imperfect AI systems can improve on existing processes.
- Promote transparency that facilitates accountability, empowering users and building trust.
Importantly, in developing new frameworks for AI, policymakers will need to reconcile contending policy objectives like competition, content moderation, privacy and security. They will also need to include mechanisms to allow rules to evolve as technology progresses. AI remains a very dynamic, fast-moving field and we will all learn from new experiences.
With a lot of collaborative, multi-stakeholder efforts already underway around the world, there’s no need to start from scratch when developing AI frameworks and responsible practices.
The U.S. National Institute of Standards and Technology AI Risk Management Framework and the OECD’s AI Principles and AI Policy Observatory are two strong examples. Developed through open and collaborative processes, they provide clear guidelines that can adapt to new AI applications, risks and developments. And we continue to provide feedback on proposals like the Europe Union’s pending AI Act.
Regulators should look first to how to use existing authorities — like rules ensuring product safety and prohibiting unlawful discrimination — pursuing new rules where they’re needed to manage truly novel challenges.
What’s next?
Whether it’s the advent of the written word or the invention of the printing press, the rise of radio or the creation of the internet, technological shifts have always brought social and economic change, and always called for new social mores and legal frameworks.
In the need to develop these norms together, AI is no different.
There are reasons for us as a society to be optimistic that thoughtful approaches and new ideas from across the AI ecosystem will help us navigate the transition, find collective solutions and maximize AI’s amazing potential. But it will take the proverbial village — collaboration and deep engagement from all of us — to get this right.
Working together on a shared agenda for progress isn’t just desirable. It’s essential.