An update on our work in responsible innovation
Over the last year, we’ve seen artificial intelligence (AI) systems advance our work in areas like inclusive product development and support for small businesses and job seekers. We’ve also seen its potential to be helpful in addressing major global needs — like forecasting and planning humanitarian responses to natural disasters, addressing global environmental challenges, and delivering groundbreaking scientific research.
AI is exciting — both from a technical perspective and when considering its underlying social benefits. And yet, to fully realize AI’s potential, it must be developed responsibly, thoughtfully and in a way that gives deep consideration to core ethical questions. After all, the promise of great reward inherently involves risk — and we’re committed to ethically developing AI in a way that is socially beneficial.
Our AI Principles guide how we integrate AI research into Google’s products and services and engage with external partners. Internally, we implement the Principles, every day, through education programs, AI ethics reviews and technical tools. There are more than 200 Googlers across the company whose full-time roles are to operationalize responsible practices for developing AI.
We’re committed to sharing our lessons learned so others across the industry can learn, too (see our posts from 2018, 2019, 2020 and 2021, and our in-depth annual AI Principles Progress Updates).
Internal education
It’s important to craft principles, but putting them into practice requires both training and constant dialogue.
Launched in late 2019, to date more than 32,000 employees across Google have engaged in AI Principles training. Given our growing understanding of effective hybrid and remote learning, we continue to expand and modify the courses. For example, this year we adapted our popular four-part Tech Ethics self-study course to a one-part deep dive based on Googler feedback. Similarly, we launched the Responsible Innovation Challenge — taken by more than 13,000 employees — as a series of engaging online puzzles, quizzes and games to raise awareness of the AI Principles and measure employees' retention of ethical concepts, such as avoiding unfair bias.
We also piloted a new Moral Imagination workshop, a two-day, live-video immersive set of activities for product teams to walk through the ethical implications of potential AI products. To date, 248 Googlers across 23 Google product and research teams have taken the workshop, resulting in deeper, ongoing AI ethics consultations on product development.
As we develop internal training, we’re committed to incorporating the input of both Googlers and outside experts. This year, when we launched a live workshop to educate our internal user experience and product teams on the concept of AI explainability, we first piloted the workshop with outside experts at the international Trust, Transparency and Control Labs summit in May.
We believe this approach complements programs like our internal AI Principles Ethics Fellows program, a six-month fellowship that this year involved Googlers from 17 different global offices. We also just launched a version of the fellowship program tailored for senior leaders.
Putting the Principles into practice
Our approach to responsible AI innovation starts early, before teams plan a new AI application. When a team starts to build a machine learning (ML) model, dataset or product feature, they can attend office hours with experts to ask questions and engage in analyses using responsible AI tools that Google develops, or seek adversarial proactive fairness (ProFair) testing. Pre-launch, a team then can request an AI Principles review.
AI Principles reviewers are in place to implement a structured assessment to identify, measure and analyze potential risk of harm. The risk rating focuses on the extent to which people and society may be impacted if solutions did not exist or were to fail. Reviewers also consider a growing body of lessons from thousands of previous AI Principles reviews conducted since 2019.
When reviewers find medium- to high-risk issues, such as product exclusion or a potential privacy or security concern, they work with the teams to address these issues. Reviews either result in an approval, approval with conditions or recommendations, or non-approval. New AI applications that might affect multiple product areas are escalated to the Advanced Technology Review Council — a group of senior research, product and business leaders who make the final decision.
To supplement the expertise of our internal AI Principles group members, we often incorporate trusted external advisors. For example, a team was incorporating AI to help build a near real-time dataset to enable reliable measurement of global land cover for environmental and social benefit. They submitted for AI Principles review and then collaborated with the review team to design several safeguards. The review team also worked with third-party experts at the World Resources Institute and BSR. Following the example of the European Commission’s Copernicus mission’s open data and services terms, the product team applied open data principles, making the ML model’s training and test data used to create the dataset, as well as the dataset itself, freely available under CC-BY-4.0, and the model available on Github under an Apache 2.0 license. We recently released a Codelab for developers to walk through the ethics review process and apply learnings to their own projects.
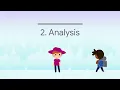
Google’s AI Principles Review Process: How we assess new AI research and applications for alignment with our Principles
Projects such as research methods for evaluating misinformation and datasets that need more diverse representation tend to receive conditions to proceed toward a launch. A recurring condition given to teams is to engage in ProFair testing with people from a diversity of backgrounds, often in partnership with our central Product Inclusion and Equity team. This year, the number of ProFair consultations increased annually by 100%. A recurring approach is to create and release detailed documentation in the form of data cards and model cards for transparency and accountability. The number of AI Principles reviews with model or data card mitigations increased 68% in the last year.
As we’ve stated, we’ve embedded customized AI governance and review committees within certain product areas (like Cloud and Health). As a result, both the Health Ethics Committee and Cloud make decisions with specialized expertise, such as establishing policies for potentially winding down the Covid-19 Community Mobility Reports and the Covid-19 Forecaster, respectively, if situations arise that might cause the data quality to degrade. This year, we extended this specialized approach and created a dedicated consumer hardware AI Principles review process.
It’s important to note that product teams across Google engage in everyday responsible AI practices even if not in formal reviews. YouTube is leveraging a more targeted mix of classifiers, keywords in additional languages, and information from regional analysts. This work is a result of collaboration with our researchers who focus on new tools for AI fairness. The Photos team participated in an Equitable AI Research Roundtable (EARR) with a group of external advisors on potential fairness considerations. And the Gboard team deployed a new, privacy-by-design approach to federated machine learning. These examples did not stem from AI Principles reviews, but reflect the adoption of the AI Principles across Google.
Tools and research
In early 2022, to offer easier access to our publications on responsible AI, we curated an external collection of more than 200 research papers focused on the topic. We continue to launch, refine and consolidate technical resources, including proactive tools like:
- The Monk Skin Tone Scale, developed by Harvard University Sociology Professor Dr. Ellis Monk. The scale offers a spectrum of skin tones from all around the world for use in evaluating and addressing fairness considerations in AI.
- The Know Your Data tool (KYD), which helps developers with tasks such as quickly identifying issues in fairness, and which has integrated the Monk Scale to help developers examine skin tone data for unfair bias.
- The Language Interpretability Tool, or LIT, to help developers probe an ML model, now with a new method to better understand, test and debug its behaviors.
- Counterfactual Logit Pairing, which helps ensure that a model’s prediction doesn’t change when sensitive attributes or identity terms referenced in an example are removed or replaced, now added to the TensorFlow Model Remediation Library (see the research paper for more).
- And to help teams measure their progress against the AI Principles, we’re piloting an internal tool to help teams assess how ML models were developed in accordance with emerging smart practices, previous reviews, and our growing body of ethics, fairness, and human-rights work.
Many responsible AI tools developed by researchers are actively in use by product teams at Google. For example, Photos, Pixel and Image Search are leveraging the Monk Skin Tone Scale.
External engagement
Ensuring the responsible development and deployment of AI is an ongoing process. We believe it should be a collaborative one, too, so we remain deeply engaged with governments across Europe, the Middle East and Africa, Latin America, Asia Pacific, and the U.S. to advocate for AI regulation that supports innovation around the world for businesses of all sizes. We share our approach to responsible AI and recommendations, comments and responses to open requests for information. We also initiated and are leading an effort with the International Standards Organization (ISO/IEC PWI TS 17866) to share best practice guidance for the development of AI.
As these efforts look toward the future, Responsible AI needs to be supported across industries today. So for current Google Cloud Partners and customers seeking best practices to help with the responsible implementation and AI governance in their organization, we added responsible AI prerequisites to the Google Cloud Partner Advantage ML Specialization, including a newly-released training, “Applying AI Principles with Google Cloud.”
To help nurture the next generation of responsible AI practitioners, we launched a free introduction to AI and machine learning for K-12 students. And we continue to develop an external Responsible Innovation Fellowship program in the U.S. for students at Historically Black Colleges and Universities (HBCUs).
Our approach to responsible innovation also means keeping an eye on emerging markets where AI is being developed. We launched a new AI research center in Bulgaria and expanded support for African entrepreneurs whose businesses use AI through our Google for Startups Accelerator: Africa.
The examples we’re sharing today are a sampling of our ongoing commitment to responsible innovation. They also reflect our ability to change and keep setting a high bar for trustworthy AI standards for our company. We remain dedicated to sharing helpful information on Google’s journey, as recommended practices for responsible AI continue to emerge and evolve.