Google I/O 2022: Advancing knowledge and computing
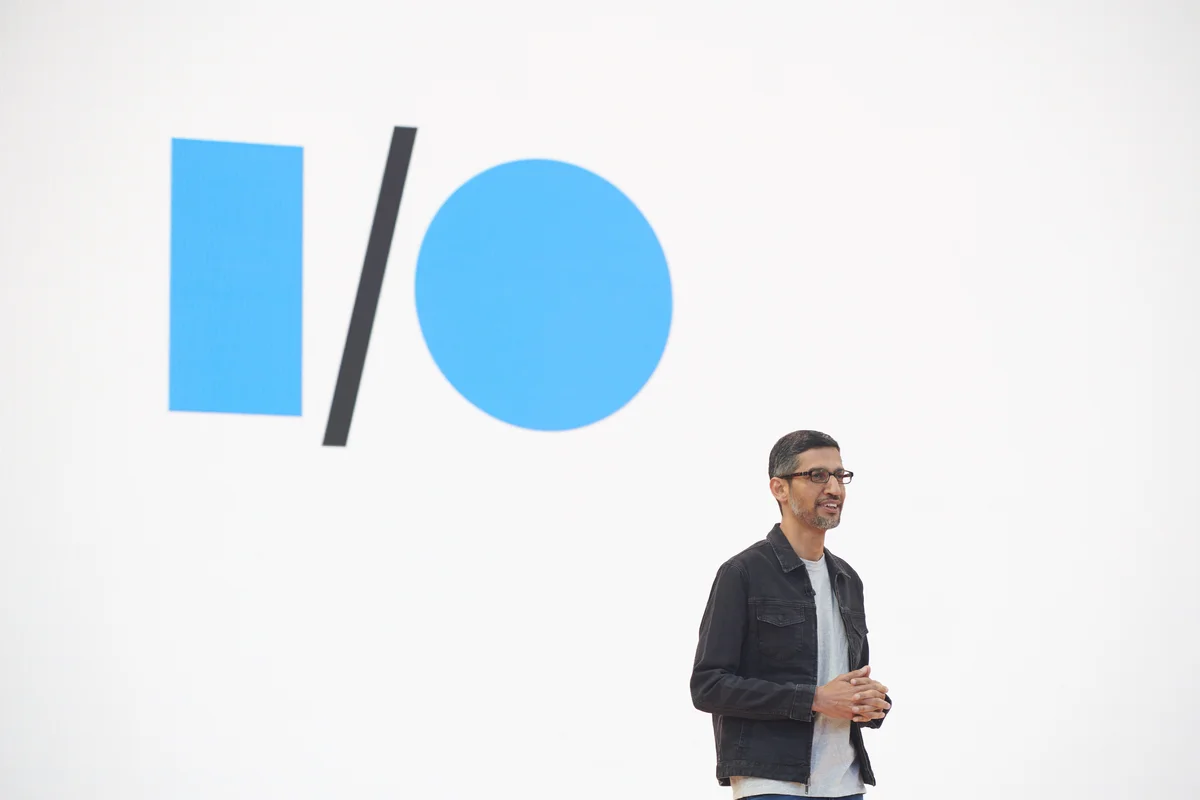
[TL;DR]
Nearly 24 years ago, Google started with two graduate students, one product, and a big mission: to organize the world’s information and make it universally accessible and useful. In the decades since, we’ve been developing our technology to deliver on that mission.
The progress we've made is because of our years of investment in advanced technologies, from AI to the technical infrastructure that powers it all. And once a year — on my favorite day of the year :) — we share an update on how it’s going at Google I/O.
Today, I talked about how we’re advancing two fundamental aspects of our mission — knowledge and computing — to create products that are built to help. It’s exciting to build these products; it’s even more exciting to see what people do with them.
Thank you to everyone who helps us do this work, and most especially our Googlers. We are grateful for the opportunity.
- Sundar
Editor’s note: Below is an edited transcript of Sundar Pichai's keynote address during the opening of today's Google I/O Developers Conference.
Hi, everyone, and welcome. Actually, let’s make that welcome back! It’s great to return to Shoreline Amphitheatre after three years away. To the thousands of developers, partners and Googlers here with us, it’s great to see all of you. And to the millions more joining us around the world — we’re so happy you’re here, too.
Last year, we shared how new breakthroughs in some of the most technically challenging areas of computer science are making Google products more helpful in the moments that matter. All this work is in service of our timeless mission: to organize the world's information and make it universally accessible and useful.
I'm excited to show you how we’re driving that mission forward in two key ways: by deepening our understanding of information so that we can turn it into knowledge; and advancing the state of computing, so that knowledge is easier to access, no matter who or where you are.
Today, you'll see how progress on these two parts of our mission ensures Google products are built to help. I’ll start with a few quick examples. Throughout the pandemic, Google has focused on delivering accurate information to help people stay healthy. Over the last year, people used Google Search and Maps to find where they could get a COVID vaccine nearly two billion times.
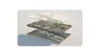
Google’s flood forecasting technology sent flood alerts to 23 million people in India and Bangladesh last year.
We’ve also expanded our flood forecasting technology to help people stay safe in the face of natural disasters. During last year’s monsoon season, our flood alerts notified more than 23 million people in India and Bangladesh. And we estimate this supported the timely evacuation of hundreds of thousands of people.
In Ukraine, we worked with the government to rapidly deploy air raid alerts. To date, we’ve delivered hundreds of millions of alerts to help people get to safety. In March I was in Poland, where millions of Ukrainians have sought refuge. Warsaw’s population has increased by nearly 20% as families host refugees in their homes, and schools welcome thousands of new students. Nearly every Google employee I spoke with there was hosting someone.
Adding 24 more languages to Google Translate
In countries around the world, Google Translate has been a crucial tool for newcomers and residents trying to communicate with one another. We’re proud of how it’s helping Ukrainians find a bit of hope and connection until they are able to return home again.
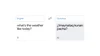
With machine learning advances, we're able to add languages like Quechua to Google Translate.
Real-time translation is a testament to how knowledge and computing come together to make people's lives better. More people are using Google Translate than ever before, but we still have work to do to make it universally accessible. There’s a long tail of languages that are underrepresented on the web today, and translating them is a hard technical problem. That’s because translation models are usually trained with bilingual text — for example, the same phrase in both English and Spanish. However, there's not enough publicly available bilingual text for every language.
So with advances in machine learning, we’ve developed a monolingual approach where the model learns to translate a new language without ever seeing a direct translation of it. By collaborating with native speakers and institutions, we found these translations were of sufficient quality to be useful, and we'll continue to improve them.
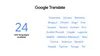
We’re adding 24 new languages to Google Translate.
Today, I’m excited to announce that we’re adding 24 new languages to Google Translate, including the first indigenous languages of the Americas. Together, these languages are spoken by more than 300 million people. Breakthroughs like this are powering a radical shift in how we access knowledge and use computers.
Taking Google Maps to the next level
So much of what’s knowable about our world goes beyond language — it’s in the physical and geospatial information all around us. For more than 15 years, Google Maps has worked to create rich and useful representations of this information to help us navigate. Advances in AI are taking this work to the next level, whether it’s expanding our coverage to remote areas, or reimagining how to explore the world in more intuitive ways.
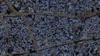
Advances in AI are helping to map remote and rural areas.
Around the world, we’ve mapped around 1.6 billion buildings and over 60 million kilometers of roads to date. Some remote and rural areas have previously been difficult to map, due to scarcity of high-quality imagery and distinct building types and terrain. To address this, we’re using computer vision and neural networks to detect buildings at scale from satellite images. As a result, we have increased the number of buildings on Google Maps in Africa by 5X since July 2020, from 60 million to nearly 300 million.
We’ve also doubled the number of buildings mapped in India and Indonesia this year. Globally, over 20% of the buildings on Google Maps have been detected using these new techniques. We’ve gone a step further, and made the dataset of buildings in Africa publicly available. International organizations like the United Nations and the World Bank are already using it to better understand population density, and to provide support and emergency assistance.
Immersive view in Google Maps fuses together aerial and street level images.
We’re also bringing new capabilities into Maps. Using advances in 3D mapping and machine learning, we’re fusing billions of aerial and street level images to create a new, high-fidelity representation of a place. These breakthrough technologies are coming together to power a new experience in Maps called immersive view: it allows you to explore a place like never before.
Let’s go to London and take a look. Say you’re planning to visit Westminster with your family. You can get into this immersive view straight from Maps on your phone, and you can pan around the sights… here’s Westminster Abbey. If you’re thinking of heading to Big Ben, you can check if there's traffic, how busy it is, and even see the weather forecast. And if you’re looking to grab a bite during your visit, you can check out restaurants nearby and get a glimpse inside.
What's amazing is that isn't a drone flying in the restaurant — we use neural rendering to create the experience from images alone. And Google Cloud Immersive Stream allows this experience to run on just about any smartphone. This feature will start rolling out in Google Maps for select cities globally later this year.
Another big improvement to Maps is eco-friendly routing. Launched last year, it shows you the most fuel-efficient route, giving you the choice to save money on gas and reduce carbon emissions. Eco-friendly routes have already rolled out in the U.S. and Canada — and people have used them to travel approximately 86 billion miles, helping save an estimated half million metric tons of carbon emissions, the equivalent of taking 100,000 cars off the road.
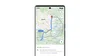
Eco-friendly routes will expand to Europe later this year.
I’m happy to share that we’re expanding this feature to more places, including Europe later this year. In this Berlin example, you could reduce your fuel consumption by 18% taking a route that’s just three minutes slower. These small decisions have a big impact at scale. With the expansion into Europe and beyond, we estimate carbon emission savings will double by the end of the year.
And we’ve added a similar feature to Google Flights. When you search for flights between two cities, we also show you carbon emission estimates alongside other information like price and schedule, making it easy to choose a greener option. These eco-friendly features in Maps and Flights are part of our goal to empower 1 billion people to make more sustainable choices through our products, and we’re excited about the progress here.
New YouTube features to help people easily access video content
Beyond Maps, video is becoming an even more fundamental part of how we share information, communicate, and learn. Often when you come to YouTube, you are looking for a specific moment in a video and we want to help you get there faster.
Last year we launched auto-generated chapters to make it easier to jump to the part you’re most interested in.
This is also great for creators because it saves them time making chapters. We’re now applying multimodal technology from DeepMind. It simultaneously uses text, audio and video to auto-generate chapters with greater accuracy and speed. With this, we now have a goal to 10X the number of videos with auto-generated chapters, from eight million today, to 80 million over the next year.
Often the fastest way to get a sense of a video’s content is to read its transcript, so we’re also using speech recognition models to transcribe videos. Video transcripts are now available to all Android and iOS users.
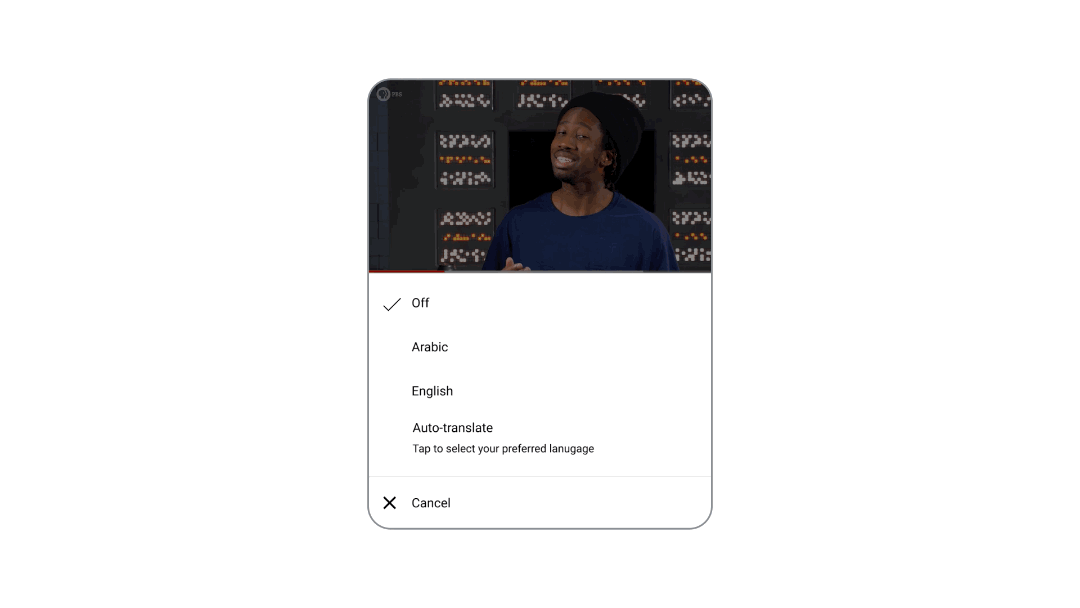
Auto-translated captions on YouTube.
Next up, we’re bringing auto-translated captions on YouTube to mobile. Which means viewers can now auto-translate video captions in 16 languages, and creators can grow their global audience. We’ll also be expanding auto-translated captions to Ukrainian YouTube content next month, part of our larger effort to increase access to accurate information about the war.
Helping people be more efficient with Google Workspace
Just as we’re using AI to improve features in YouTube, we’re building it into our Workspace products to help people be more efficient. Whether you work for a small business or a large institution, chances are you spend a lot of time reading documents. Maybe you’ve felt that wave of panic when you realize you have a 25-page document to read ahead of a meeting that starts in five minutes.
At Google, whenever I get a long document or email, I look for a TL;DR at the top — TL;DR is short for “Too Long, Didn’t Read.” And it got us thinking, wouldn’t life be better if more things had a TL;DR?
That’s why we’ve introduced automated summarization for Google Docs. Using one of our machine learning models for text summarization, Google Docs will automatically parse the words and pull out the main points.
This marks a big leap forward for natural language processing. Summarization requires understanding of long passages, information compression and language generation, which used to be outside of the capabilities of even the best machine learning models.
And docs are only the beginning. We’re launching summarization for other products in Workspace. It will come to Google Chat in the next few months, providing a helpful digest of chat conversations, so you can jump right into a group chat or look back at the key highlights.
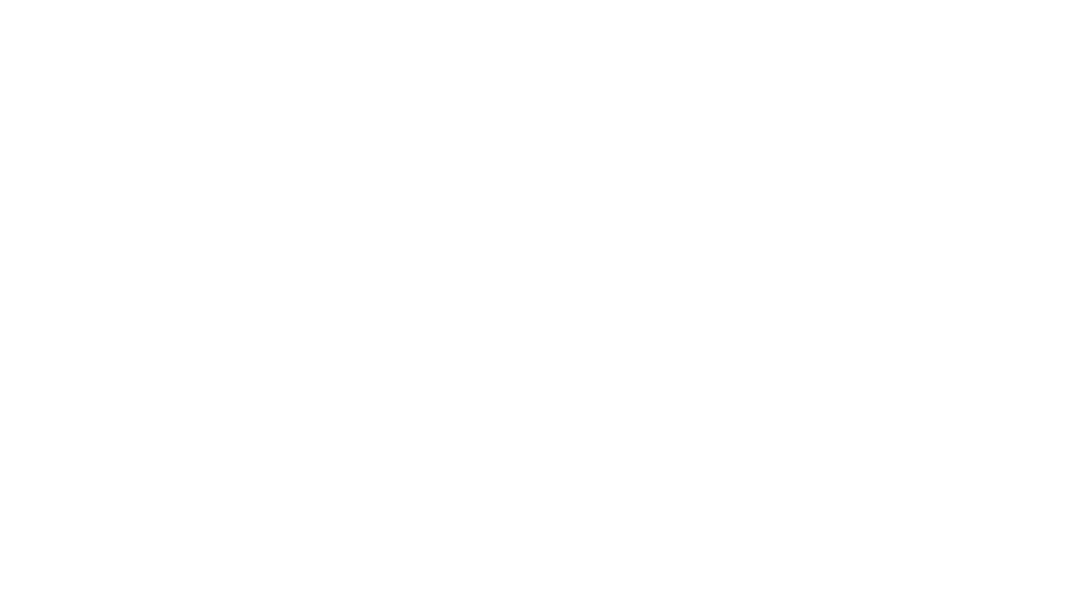
We’re bringing summarization to Google Chat in the coming months.
And we’re working to bring transcription and summarization to Google Meet as well so you can catch up on some important meetings you missed.
Visual improvements on Google Meet
Of course there are many moments where you really want to be in a virtual room with someone. And that’s why we continue to improve audio and video quality, inspired by Project Starline. We introduced Project Starline at I/O last year. And we’ve been testing it across Google offices to get feedback and improve the technology for the future. And in the process, we’ve learned some things that we can apply right now to Google Meet.
Starline inspired machine learning-powered image processing to automatically improve your image quality in Google Meet. And it works on all types of devices so you look your best wherever you are.
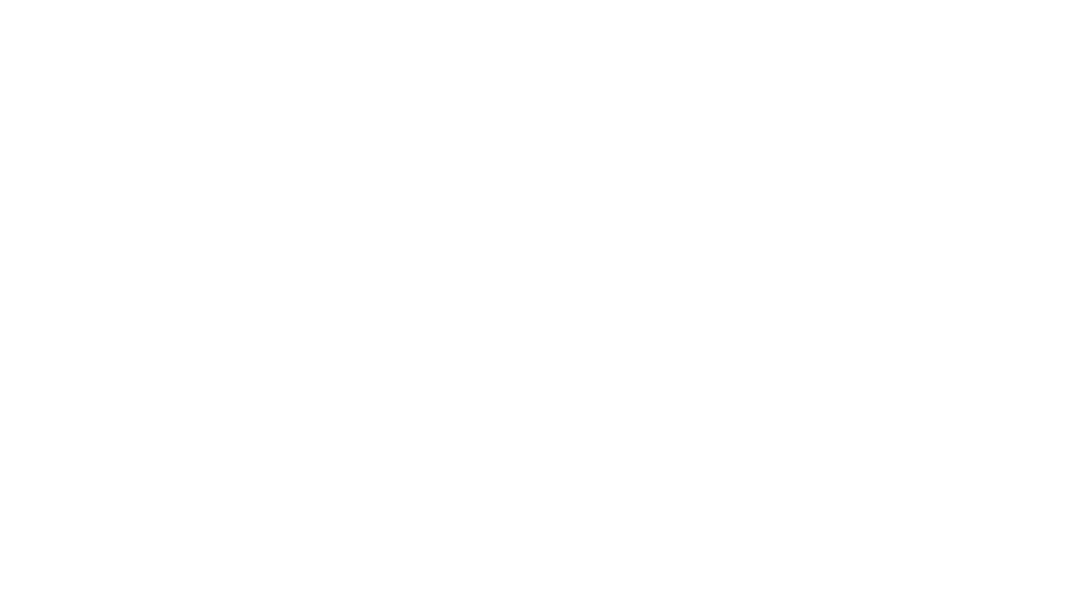
Machine learning-powered image processing automatically improves image quality in Google Meet.
We’re also bringing studio quality virtual lighting to Meet. You can adjust the light position and brightness, so you’ll still be visible in a dark room or sitting in front of a window. We’re testing this feature to ensure everyone looks like their true selves, continuing the work we’ve done with Real Tone on Pixel phones and the Monk Scale.
These are just some of the ways AI is improving our products: making them more helpful, more accessible, and delivering innovative new features for everyone.
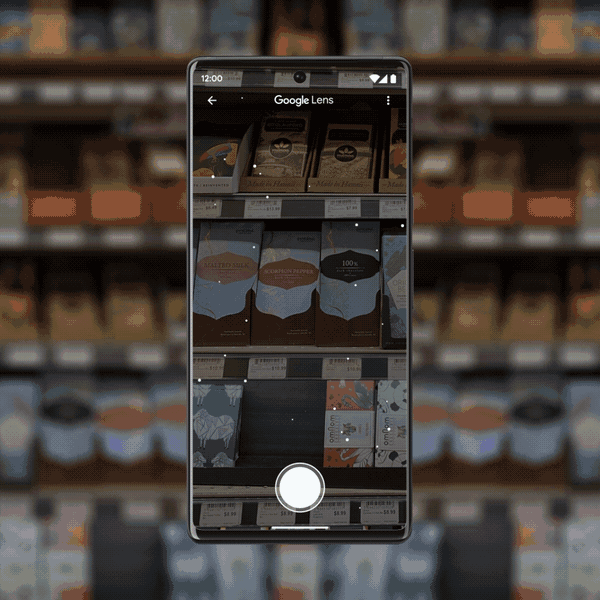
Today at I/O Prabhakar Raghavan shared how we’re helping people find helpful information in more intuitive ways on Search.
Making knowledge accessible through computing
We’ve talked about how we’re advancing access to knowledge as part of our mission: from better language translation to improved Search experiences across images and video, to richer explorations of the world using Maps.
Now we’re going to focus on how we make that knowledge even more accessible through computing. The journey we’ve been on with computing is an exciting one. Every shift, from desktop to the web to mobile to wearables and ambient computing has made knowledge more useful in our daily lives.
As helpful as our devices are, we’ve had to work pretty hard to adapt to them. I’ve always thought computers should be adapting to people, not the other way around. We continue to push ourselves to make progress here.
Here’s how we’re making computing more natural and intuitive with the Google Assistant.
Introducing LaMDA 2 and AI Test Kitchen
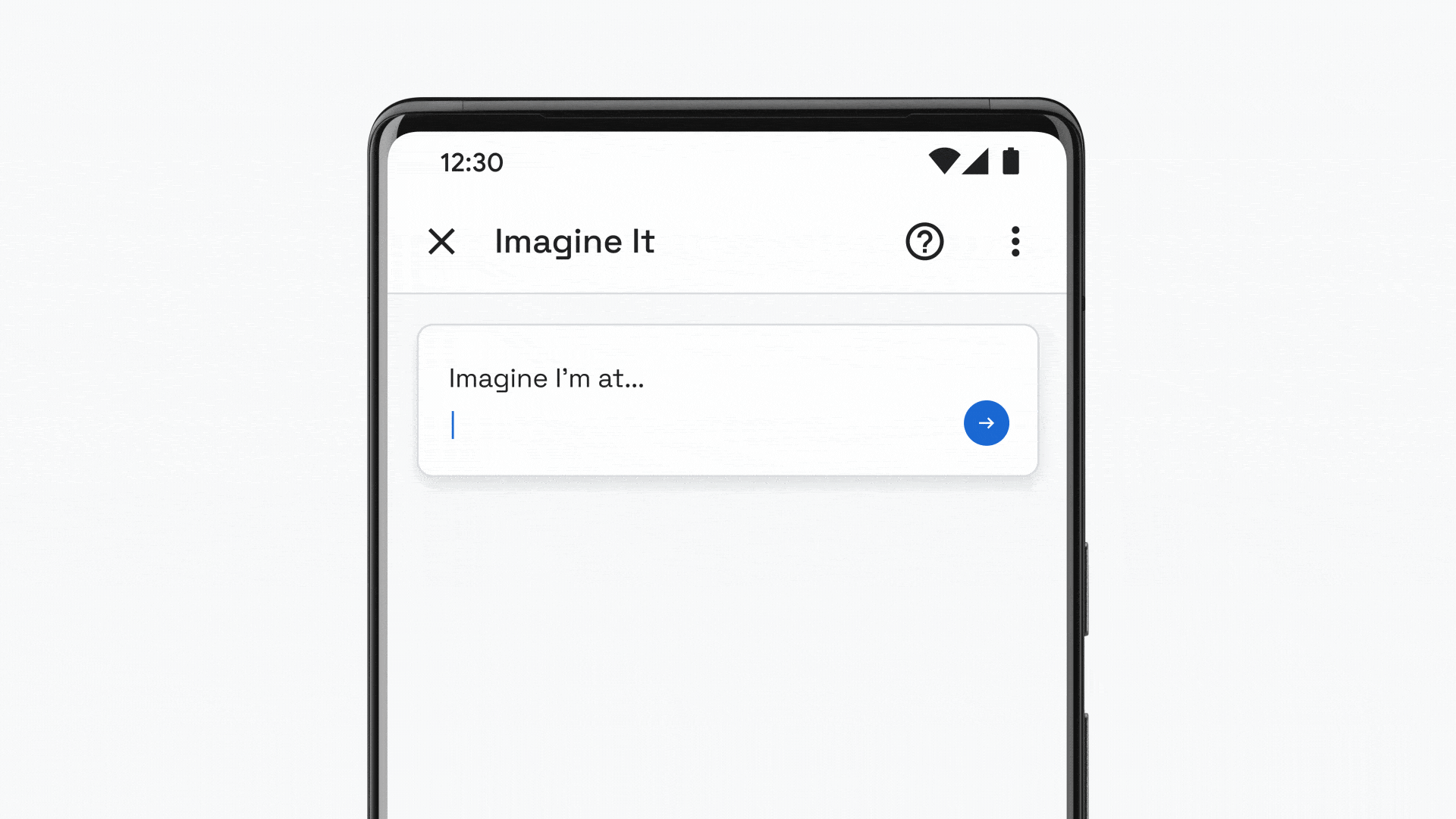
A demo of LaMDA, our generative language model for dialogue application, and the AI Test Kitchen.
We're continually working to advance our conversational capabilities. Conversation and natural language processing are powerful ways to make computers more accessible to everyone. And large language models are key to this.
Last year, we introduced LaMDA, our generative language model for dialogue applications that can converse on any topic. Today, we are excited to announce LaMDA 2, our most advanced conversational AI yet.
We are at the beginning of a journey to make models like these useful to people, and we feel a deep responsibility to get it right. To make progress, we need people to experience the technology and provide feedback. We opened LaMDA up to thousands of Googlers, who enjoyed testing it and seeing its capabilities. This yielded significant quality improvements, and led to a reduction in inaccurate or offensive responses.
That’s why we’ve made AI Test Kitchen. It’s a new way to explore AI features with a broader audience. Inside the AI Test Kitchen, there are a few different experiences. Each is meant to give you a sense of what it might be like to have LaMDA in your hands and use it for things you care about.
The first is called “Imagine it.” This demo tests if the model can take a creative idea you give it, and generate imaginative and relevant descriptions. These are not products, they are quick sketches that allow us to explore what LaMDA can do with you. The user interfaces are very simple.
Say you’re writing a story and need some inspirational ideas. Maybe one of your characters is exploring the deep ocean. You can ask what that might feel like. Here LaMDA describes a scene in the Mariana Trench. It even generates follow-up questions on the fly. You can ask LaMDA to imagine what kinds of creatures might live there. Remember, we didn’t hand-program the model for specific topics like submarines or bioluminescence. It synthesized these concepts from its training data. That’s why you can ask about almost any topic: Saturn’s rings or even being on a planet made of ice cream.
Staying on topic is a challenge for language models. Say you’re building a learning experience — you want it to be open-ended enough to allow people to explore where curiosity takes them, but stay safely on topic. Our second demo tests how LaMDA does with that.
In this demo, we’ve primed the model to focus on the topic of dogs. It starts by generating a question to spark conversation, “Have you ever wondered why dogs love to play fetch so much?” And if you ask a follow-up question, you get an answer with some relevant details: it’s interesting, it thinks it might have something to do with the sense of smell and treasure hunting.
You can take the conversation anywhere you want. Maybe you’re curious about how smell works and you want to dive deeper. You’ll get a unique response for that too. No matter what you ask, it will try to keep the conversation on the topic of dogs. If I start asking about cricket, which I probably would, the model brings the topic back to dogs in a fun way.
This challenge of staying on-topic is a tricky one, and it’s an important area of research for building useful applications with language models.
These experiences show the potential of language models to one day help us with things like planning, learning about the world, and more.
Of course, there are significant challenges to solve before these models can truly be useful. While we have improved safety, the model might still generate inaccurate, inappropriate, or offensive responses. That’s why we are inviting feedback in the app, so people can help report problems.
We will be doing all of this work in accordance with our AI Principles. Our process will be iterative, opening up access over the coming months, and carefully assessing feedback with a broad range of stakeholders — from AI researchers and social scientists to human rights experts. We’ll incorporate this feedback into future versions of LaMDA, and share our findings as we go.
Over time, we intend to continue adding other emerging areas of AI into AI Test Kitchen. You can learn more at: g.co/AITestKitchen.
Advancing AI language models
LaMDA 2 has incredible conversational capabilities. To explore other aspects of natural language processing and AI, we recently announced a new model. It’s called Pathways Language Model, or PaLM for short. It’s our largest model to date and trained on 540 billion parameters.
PaLM demonstrates breakthrough performance on many natural language processing tasks, such as generating code from text, answering a math word problem, or even explaining a joke.
It achieves this through greater scale. And when we combine that scale with a new technique called chain-of- thought prompting, the results are promising. Chain-of-thought prompting allows us to describe multi-step problems as a series of intermediate steps.
Let’s take an example of a math word problem that requires reasoning. Normally, how you use a model is you prompt it with a question and answer, and then you start asking questions. In this case: How many hours are in the month of May? So you can see, the model didn’t quite get it right.
In chain-of-thought prompting, we give the model a question-answer pair, but this time, an explanation of how the answer was derived. Kind of like when your teacher gives you a step-by-step example to help you understand how to solve a problem. Now, if we ask the model again — how many hours are in the month of May — or other related questions, it actually answers correctly and even shows its work.
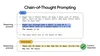
Chain-of-thought prompting leads to better reasoning and more accurate answers.
Chain-of-thought prompting increases accuracy by a large margin. This leads to state-of-the-art performance across several reasoning benchmarks, including math word problems. And we can do it all without ever changing how the model is trained.
PaLM is highly capable and can do so much more. For example, you might be someone who speaks a language that’s not well-represented on the web today — which makes it hard to find information. Even more frustrating because the answer you are looking for is probably out there. PaLM offers a new approach that holds enormous promise for making knowledge more accessible for everyone.
Let me show you an example in which we can help answer questions in a language like Bengali — spoken by a quarter billion people. Just like before we prompt the model with two examples of questions in Bengali with both Bengali and English answers.
That’s it, now we can start asking questions in Bengali: “What is the national song of Bangladesh?” The answer, by the way, is “Amar Sonar Bangla” — and PaLM got it right, too. This is not that surprising because you would expect that content to exist in Bengali.
You can also try something that is less likely to have related information in Bengali such as: “What are popular pizza toppings in New York City?” The model again answers correctly in Bengali. Though it probably just stirred up a debate amongst New Yorkers about how “correct” that answer really is.
What’s so impressive is that PaLM has never seen parallel sentences between Bengali and English. Nor was it ever explicitly taught to answer questions or translate at all! The model brought all of its capabilities together to answer questions correctly in Bengali. And we can extend the techniques to more languages and other complex tasks.
We're so optimistic about the potential for language models. One day, we hope we can answer questions on more topics in any language you speak, making knowledge even more accessible, in Search and across all of Google.
Introducing the world’s largest, publicly available machine learning hub
The advances we’ve shared today are possible only because of our continued innovation in our infrastructure. Recently we announced plans to invest $9.5 billion in data centers and offices across the U.S.
One of our state-of-the-art data centers is in Mayes County, Oklahoma. I’m excited to announce that, there, we are launching the world’s largest, publicly-available machine learning hub for our Google Cloud customers.
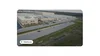
One of our state-of-the-art data centers in Mayes County, Oklahoma.
This machine learning hub has eight Cloud TPU v4 pods, custom-built on the same networking infrastructure that powers Google’s largest neural models. They provide nearly nine exaflops of computing power in aggregate — bringing our customers an unprecedented ability to run complex models and workloads. We hope this will fuel innovation across many fields, from medicine to logistics, sustainability and more.
And speaking of sustainability, this machine learning hub is already operating at 90% carbon-free energy. This is helping us make progress on our goal to become the first major company to operate all of our data centers and campuses globally on 24/7 carbon-free energy by 2030.
Even as we invest in our data centers, we are working to innovate on our mobile platforms so more processing can happen locally on device. Google Tensor, our custom system on a chip, was an important step in this direction. It’s already running on Pixel 6 and Pixel 6 Pro, and it brings our AI capabilities — including the best speech recognition we’ve ever deployed — right to your phone. It’s also a big step forward in making those devices more secure. Combined with Android’s Private Compute Core, it can run data-powered features directly on device so that it’s private to you.
People turn to our products every day for help in moments big and small. Core to making this possible is protecting your private information each step of the way. Even as technology grows increasingly complex, we keep more people safe online than anyone else in the world, with products that are secure by default, private by design and that put you in control.
We also spent time today sharing updates to platforms like Android. They’re delivering access, connectivity, and information to billions of people through their smartphones and other connected devices like TVs, cars and watches.
And we shared our new Pixel Portfolio, including the Pixel 6a, Pixel Buds Pro, Google Pixel Watch, Pixel 7, and Pixel tablet all built with ambient computing in mind. We’re excited to share a family of devices that work better together — for you.
The next frontier of computing: augmented reality
Today we talked about all the technologies that are changing how we use computers and access knowledge. We see devices working seamlessly together, exactly when and where you need them and with conversational interfaces that make it easier to get things done.
Looking ahead, there's a new frontier of computing, which has the potential to extend all of this even further, and that is augmented reality. At Google, we have been heavily invested in this area. We’ve been building augmented reality into many Google products, from Google Lens to multisearch, scene exploration, and Live and immersive views in Maps.
These AR capabilities are already useful on phones and the magic will really come alive when you can use them in the real world without the technology getting in the way.
That potential is what gets us most excited about AR: the ability to spend time focusing on what matters in the real world, in our real lives. Because the real world is pretty amazing!
It’s important we design in a way that is built for the real world — and doesn’t take you away from it. And AR gives us new ways to accomplish this.
Let’s take language as an example. Language is just so fundamental to connecting with one another. And yet, understanding someone who speaks a different language, or trying to follow a conversation if you are deaf or hard of hearing can be a real challenge. Let's see what happens when we take our advancements in translation and transcription and deliver them in your line of sight in one of the early prototypes we’ve been testing.
You can see it in their faces: the joy that comes with speaking naturally to someone. That moment of connection. To understand and be understood. That’s what our focus on knowledge and computing is all about. And it’s what we strive for every day, with products that are built to help.
Each year we get a little closer to delivering on our timeless mission. And we still have so much further to go. At Google, we genuinely feel a sense of excitement about that. And we are optimistic that the breakthroughs you just saw will help us get there. Thank you to all of the developers, partners and customers who joined us today. We look forward to building the future with all of you.